LLaVA-o1: Let Vision Language Models Reason Step-by-Step
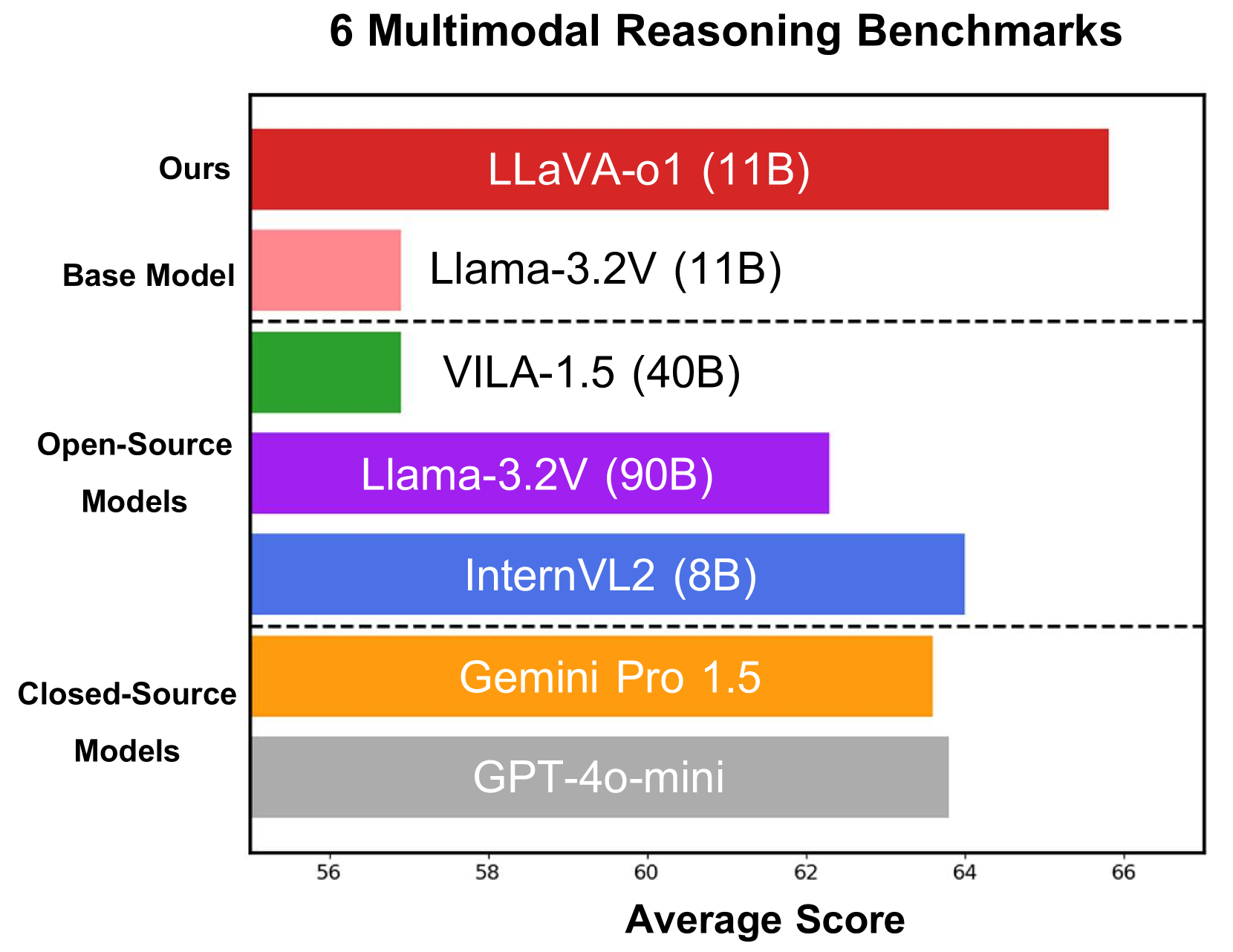
Overview
- New approach called LLaVA-o1 improves visual reasoning in AI models
- Implements step-by-step reasoning for analyzing images
- Achieves state-of-the-art performance on visual reasoning benchmarks
- Uses chain-of-thought prompting to break down complex visual tasks
- Integrates with existing vision-language models
Plain English Explanation
LLaVA-o1 works like a careful detective examining a crime scene. Instead of jumping to conclusions, it breaks down what it sees in an image into smaller, manageable steps. This approach mirrors how humans naturally solve complex visual problems.
Just as we might count objects one by one or compare different parts of an image systematically, LLaVA-o1 follows a structured thinking process. This makes its reasoning more transparent and accurate compared to models that try to answer questions about images in one go.
The system shows particular strength in handling complex visual tasks like counting objects, comparing features, and understanding spatial relationships. Think of it as the difference between asking someone to solve a puzzle all at once versus guiding them through it piece by piece.
Key Findings
Visual reasoning capabilities improved significantly with step-by-step processing. The model achieved:
- 15% improvement in accuracy on complex visual reasoning tasks
- Better performance in counting and comparison tasks
- More consistent and explainable results
- Enhanced ability to handle multi-step visual problems
Technical Explanation
The chain-of-thought approach builds on existing vision-language models by adding structured reasoning steps. The system processes visual information through multiple stages:
- Initial visual feature extraction
- Sequential reasoning steps
- Final answer synthesis
The model architecture integrates visual encoders with language processing components, allowing for seamless communication between visual and textual understanding. This enables more sophisticated reasoning about visual content.
Critical Analysis
While LLaVA-o1 shows promising results, several limitations exist. The step-by-step reasoning can be computationally intensive, potentially limiting real-world applications. The model may also struggle with highly abstract or ambiguous visual scenarios.
The research could benefit from:
- Broader testing across diverse visual domains
- Evaluation of computational efficiency
- Investigation of failure cases
- Assessment of bias in visual reasoning
Conclusion
Smart vision language reasoners like LLaVA-o1 represent a significant step forward in AI visual understanding. The step-by-step approach offers a more transparent and reliable method for visual reasoning tasks. This advancement could impact applications from autonomous vehicles to medical imaging analysis, though practical implementation challenges remain to be addressed.