Medical Video Generation for Disease Progression Simulation
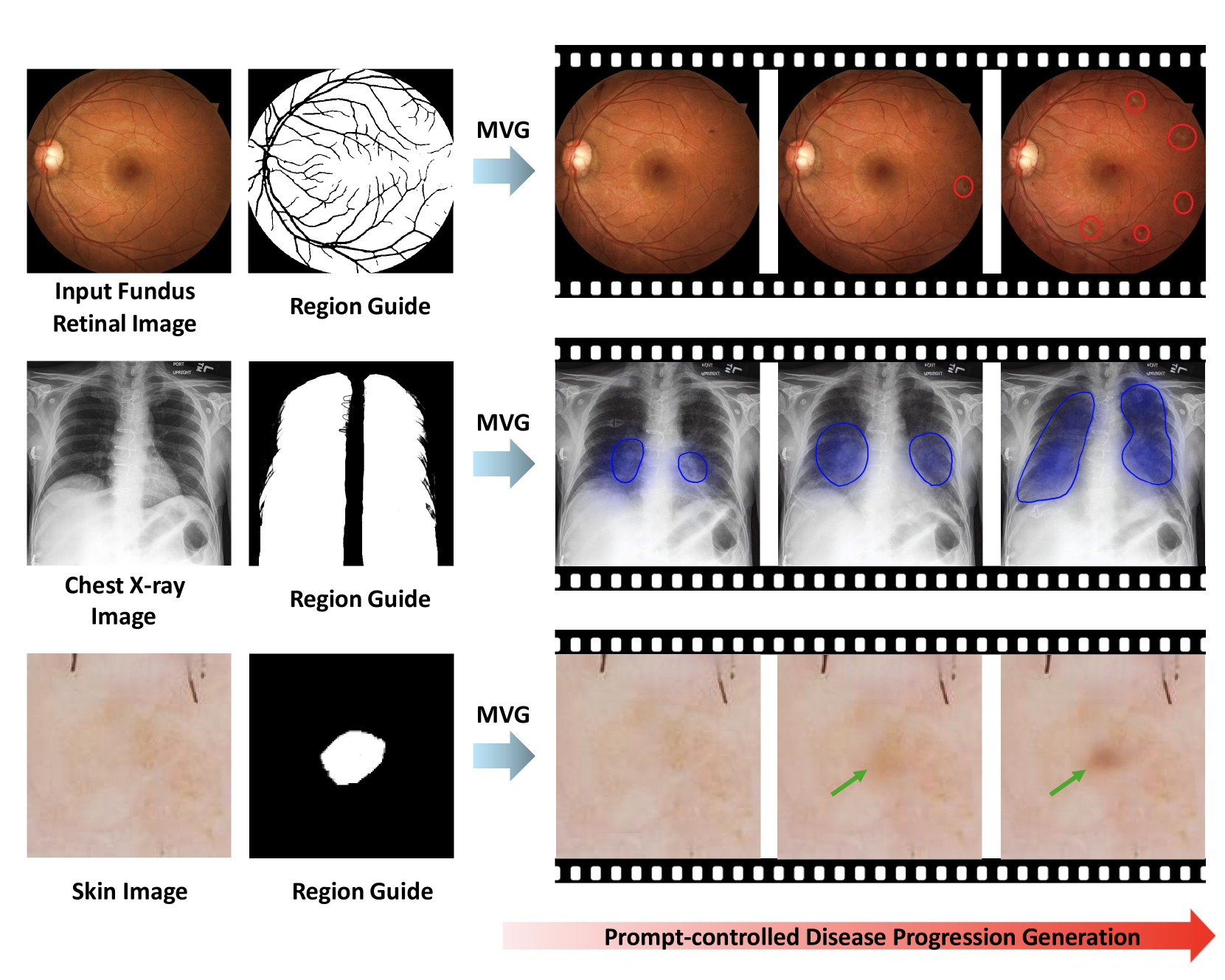
Overview
- New AI system generates realistic medical videos showing disease progression
- Combines text-to-video generation with medical imaging expertise
- Creates temporal sequences showing how conditions evolve
- Validated on retinal disease progression
- Maintains clinical accuracy while generating novel sequences
Plain English Explanation
This research introduces a new way to create medical videos that show how diseases develop over time. Think of it like a weather forecast simulation, but instead of showing how clouds move, it shows how medical conditions progress in the body.
The system takes medical imaging data and written descriptions as input. It then generates realistic video sequences showing disease progression. For example, it can create a video showing how an eye condition develops over months or years.
This tool could help doctors train, plan treatments, and explain conditions to patients. Instead of just showing static before-and-after images, doctors can now demonstrate the full progression of a disease.
Key Findings
The researchers demonstrated their system using retinal disease data:
- Generated videos matched real disease progression patterns
- Medical experts validated the clinical accuracy
- System maintained consistent patient-specific features
- Videos showed smooth, realistic transitions between disease stages
- Disease progression simulation maintained anatomical correctness
Technical Explanation
The system uses a two-stage architecture. First, it generates key frames representing major progression points. Then it creates smooth transitions between these frames.
The model incorporates conditional diffusion techniques to ensure generated sequences follow realistic progression patterns. It maintains patient-specific features while showing disease development.
Training used paired sequences of retinal images with corresponding clinical descriptions. The system learned to map text descriptions to appropriate visual progression patterns.
Critical Analysis
Limitations include:
- Currently only validated on retinal diseases
- Requires large datasets of sequential medical images
- Cannot guarantee accuracy for rare disease variants
- May not capture all possible progression paths
Further research should explore applications to other medical imaging types and validation across more diverse patient populations.
Conclusion
This breakthrough in biomedical video generation could transform medical education and patient communication. The ability to visualize disease progression helps bridge understanding between medical professionals and patients.
The technology shows promise for expanding to other medical domains beyond ophthalmology. As the system develops, it could become a valuable tool for training new doctors and planning personalized treatments.