Marqo Ecommerce Embedding Models
In this work, we introduce two state-of-the-art embedding models for ecommerce products: Marqo-Ecommerce-B and Marqo-Ecommerce-L.
The benchmarking results show that the Marqo-Ecommerce models consistently outperformed all other models across various metrics. Specifically, marqo-ecommerce-L
achieved an average improvement of 17.6% in MRR and 20.5% in nDCG@10 when compared with the current best open source model, ViT-SO400M-14-SigLIP
across all three tasks in the marqo-ecommerce-hard
dataset. When compared with the best private model, Amazon-Titan-Multimodal
, we saw an average improvement of 38.9% in MRR and 45.1% in nDCG@10 across all three tasks, and 35.9% in Recall across the Text-to-Image tasks in the marqo-ecommerce-hard
dataset.
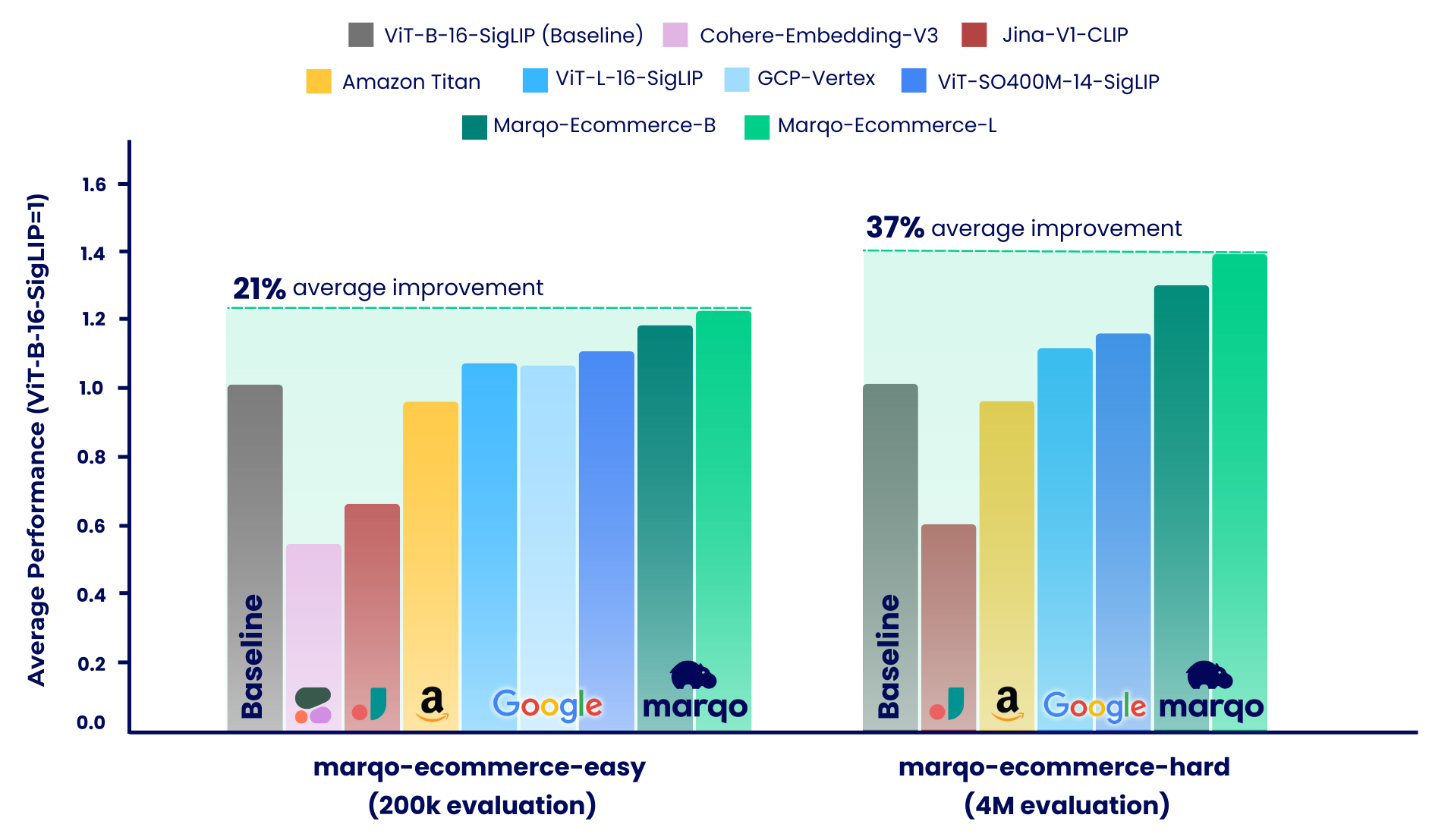
More benchmarking results can be found below.
Released Content:
- Marqo-Ecommerce-B and Marqo-Ecommerce-L embedding models
- GoogleShopping-1m and AmazonProducts-3m for evaluation
- Evaluation Code
Models
Embedding Model | #Params (m) | Dimension | HuggingFace | Download .pt |
---|---|---|---|---|
Marqo-Ecommerce-B | 203 | 768 | Marqo/marqo-ecommerce-embeddings-B | link |
Marqo-Ecommerce-L | 652 | 1024 | Marqo/marqo-ecommerce-embeddings-L | link |
Load from HuggingFace with transformers
To load the models in Transformers, see below. The models are hosted on Hugging Face and loaded using Transformers.
from transformers import AutoModel, AutoProcessor
import torch
from PIL import Image
import requests
model_name= 'Marqo/marqo-ecommerce-embeddings-B'
# model_name = 'Marqo/marqo-ecommerce-embeddings-L'
model = AutoModel.from_pretrained(model_name, trust_remote_code=True)
processor = AutoProcessor.from_pretrained(model_name, trust_remote_code=True)
img = Image.open(requests.get('https://raw.githubusercontent.com/marqo-ai/marqo-ecommerce-embeddings/refs/heads/main/images/dining-chairs.png', stream=True).raw).convert("RGB")
image = [img]
text = ["dining chairs", "a laptop", "toothbrushes"]
processed = processor(text=text, images=image, padding='max_length', return_tensors="pt")
processor.image_processor.do_rescale = False
with torch.no_grad():
image_features = model.get_image_features(processed['pixel_values'], normalize=True)
text_features = model.get_text_features(processed['input_ids'], normalize=True)
text_probs = (100 * image_features @ text_features.T).softmax(dim=-1)
print(text_probs)
# [1.0000e+00, 8.3131e-12, 5.2173e-12]
Load from HuggingFace with OpenCLIP
To load the models in OpenCLIP, see below. The models are hosted on Hugging Face and loaded using OpenCLIP. You can also find this code inside run_models.py
.
pip install open_clip_torch
from PIL import Image
import open_clip
import requests
import torch
# Specify model from Hugging Face Hub
model_name = 'hf-hub:Marqo/marqo-ecommerce-embeddings-B'
# model_name = 'hf-hub:Marqo/marqo-ecommerce-embeddings-L'
model, preprocess_train, preprocess_val = open_clip.create_model_and_transforms(model_name)
tokenizer = open_clip.get_tokenizer(model_name)
# Preprocess the image and tokenize text inputs
# Load an example image from a URL
img = Image.open(requests.get('https://raw.githubusercontent.com/marqo-ai/marqo-ecommerce-embeddings/refs/heads/main/images/dining-chairs.png', stream=True).raw)
image = preprocess_val(img).unsqueeze(0)
text = tokenizer(["dining chairs", "a laptop", "toothbrushes"])
# Perform inference
with torch.no_grad(), torch.cuda.amp.autocast():
image_features = model.encode_image(image, normalize=True)
text_features = model.encode_text(text, normalize=True)
# Calculate similarity probabilities
text_probs = (100.0 * image_features @ text_features.T).softmax(dim=-1)
# Display the label probabilities
print("Label probs:", text_probs)
# [1.0000e+00, 8.3131e-12, 5.2173e-12]
Evaluation
Generalised Contrastiove Learning (GCL) is used for the evaluation. The following code can also be found in scripts
.
git clone https://github.com/marqo-ai/GCL
Install the packages required by GCL.
1. GoogleShopping-Text2Image Retrieval.
cd ./GCL
MODEL=hf-hub:Marqo/marqo-ecommerce-B
outdir=MarqoModels/GE/marqo-ecommerce-B/gs-title2image
mkdir -p $outdir
hfdataset=Marqo/google-shopping-general-eval
python evals/eval_hf_datasets_v1.py \
--model_name $MODEL \
--hf-dataset $hfdataset \
--output-dir $outdir \
--batch-size 1024 \
--num_workers 8 \
--left-key "['title']" \
--right-key "['image']" \
--img-or-txt "[['txt'], ['img']]" \
--left-weight "[1]" \
--right-weight "[1]" \
--run-queries-cpu \
--top-q 4000 \
--doc-id-key item_ID \
--context-length "[[64], [0]]"
2. GoogleShopping-Category2Image Retrieval.
cd ./GCL
MODEL=hf-hub:Marqo/marqo-ecommerce-B
outdir=MarqoModels/GE/marqo-ecommerce-B/gs-cat2image
mkdir -p $outdir
hfdataset=Marqo/google-shopping-general-eval
python evals/eval_hf_datasets_v1.py \
--model_name $MODEL \
--hf-dataset $hfdataset \
--output-dir $outdir \
--batch-size 1024 \
--num_workers 8 \
--left-key "['query']" \
--right-key "['image']" \
--img-or-txt "[['txt'], ['img']]" \
--left-weight "[1]" \
--right-weight "[1]" \
--run-queries-cpu \
--top-q 4000 \
--doc-id-key item_ID \
--context-length "[[64], [0]]"
3. AmazonProducts-Category2Image Retrieval.
cd ./GCL
MODEL=hf-hub:Marqo/marqo-ecommerce-B
outdir=MarqoModels/GE/marqo-ecommerce-B/ap-title2image
mkdir -p $outdir
hfdataset=Marqo/amazon-products-eval
python evals/eval_hf_datasets_v1.py \
--model_name $MODEL \
--hf-dataset $hfdataset \
--output-dir $outdir \
--batch-size 1024 \
--num_workers 8 \
--left-key "['title']" \
--right-key "['image']" \
--img-or-txt "[['txt'], ['img']]" \
--left-weight "[1]" \
--right-weight "[1]" \
--run-queries-cpu \
--top-q 4000 \
--doc-id-key item_ID \
--context-length "[[64], [0]]"
Detailed Performance
Our benchmarking process was divided into two distinct regimes, each using different datasets of ecommerce product listings: marqo-ecommerce-hard and marqo-ecommerce-easy. Both datasets contained product images and text and only differed in size. The "easy" dataset is approximately 10-30 times smaller (200k vs 4M products), and designed to accommodate rate-limited models, specifically Cohere-Embeddings-v3 and GCP-Vertex (with limits of 0.66 rps and 2 rps respectively). The "hard" dataset represents the true challenge, since it contains four million ecommerce product listings and is more representative of real-world ecommerce search scenarios.
Within both these scenarios, the models were benchmarked against three different tasks:
- Google Shopping Text-to-Image
- Google Shopping Category-to-Image
- Amazon Products Text-to-Image
Marqo-Ecommerce-Hard
Marqo-Ecommerce-Hard looks into the comprehensive evaluation conducted using the full 4 million dataset, highlighting the robust performance of our models in a real-world context.
GoogleShopping-Text2Image Retrieval.
Embedding Model | mAP | R@10 | MRR | nDCG@10 |
---|---|---|---|---|
Marqo-Ecommerce-L | 0.682 | 0.878 | 0.683 | 0.726 |
Marqo-Ecommerce-B | 0.623 | 0.832 | 0.624 | 0.668 |
ViT-SO400M-14-SigLip | 0.573 | 0.763 | 0.574 | 0.613 |
ViT-L-16-SigLip | 0.540 | 0.722 | 0.540 | 0.577 |
ViT-B-16-SigLip | 0.476 | 0.660 | 0.477 | 0.513 |
Amazon-Titan-MultiModal | 0.475 | 0.648 | 0.475 | 0.509 |
Jina-V1-CLIP | 0.285 | 0.402 | 0.285 | 0.306 |
GoogleShopping-Category2Image Retrieval.
Embedding Model | mAP | P@10 | MRR | nDCG@10 |
---|---|---|---|---|
Marqo-Ecommerce-L | 0.463 | 0.652 | 0.822 | 0.666 |
Marqo-Ecommerce-B | 0.423 | 0.629 | 0.810 | 0.644 |
ViT-SO400M-14-SigLip | 0.352 | 0.516 | 0.707 | 0.529 |
ViT-L-16-SigLip | 0.324 | 0.497 | 0.687 | 0.509 |
ViT-B-16-SigLip | 0.277 | 0.458 | 0.660 | 0.473 |
Amazon-Titan-MultiModal | 0.246 | 0.429 | 0.642 | 0.446 |
Jina-V1-CLIP | 0.123 | 0.275 | 0.504 | 0.294 |
AmazonProducts-Text2Image Retrieval.
Embedding Model | mAP | R@10 | MRR | nDCG@10 |
---|---|---|---|---|
Marqo-Ecommerce-L | 0.658 | 0.854 | 0.663 | 0.703 |
Marqo-Ecommerce-B | 0.592 | 0.795 | 0.597 | 0.637 |
ViT-SO400M-14-SigLip | 0.560 | 0.742 | 0.564 | 0.599 |
ViT-L-16-SigLip | 0.544 | 0.715 | 0.548 | 0.580 |
ViT-B-16-SigLip | 0.480 | 0.650 | 0.484 | 0.515 |
Amazon-Titan-MultiModal | 0.456 | 0.627 | 0.457 | 0.491 |
Jina-V1-CLIP | 0.265 | 0.378 | 0.266 | 0.285 |
Marqo-Ecommerce-Easy
As mentioned, our benchmarking process was divided into two distinct scenarios: marqo-ecommerce-hard and marqo-ecommerce-easy. This section covers the latter which features a corpus 10-30 times smaller and was designed to accommodate rate-limited models. We will look into the comprehensive evaluation conducted using the full 200k products across the two datasets. In addition to the models already benchmarked above, these benchmarks also include Cohere-embedding-v3 and GCP-Vertex.
GoogleShopping-Text2Image Retrieval.
Embedding Model | mAP | R@10 | MRR | nDCG@10 |
---|---|---|---|---|
Marqo-Ecommerce-L | 0.879 | 0.971 | 0.879 | 0.901 |
Marqo-Ecommerce-B | 0.842 | 0.961 | 0.842 | 0.871 |
ViT-SO400M-14-SigLip | 0.792 | 0.935 | 0.792 | 0.825 |
GCP-Vertex | 0.740 | 0.910 | 0.740 | 0.779 |
ViT-L-16-SigLip | 0.754 | 0.907 | 0.754 | 0.789 |
ViT-B-16-SigLip | 0.701 | 0.870 | 0.701 | 0.739 |
Amazon-Titan-MultiModal | 0.694 | 0.868 | 0.693 | 0.733 |
Jina-V1-CLIP | 0.480 | 0.638 | 0.480 | 0.511 |
Cohere-embedding-v3 | 0.358 | 0.515 | 0.358 | 0.389 |
GoogleShopping-Category2Image Retrieval.
Embedding Model | mAP | P@10 | MRR | nDCG@10 |
---|---|---|---|---|
Marqo-Ecommerce-L | 0.515 | 0.358 | 0.764 | 0.590 |
Marqo-Ecommerce-B | 0.479 | 0.336 | 0.744 | 0.558 |
ViT-SO400M-14-SigLip | 0.423 | 0.302 | 0.644 | 0.487 |
GCP-Vertex | 0.417 | 0.298 | 0.636 | 0.481 |
ViT-L-16-SigLip | 0.392 | 0.281 | 0.627 | 0.458 |
ViT-B-16-SigLip | 0.347 | 0.252 | 0.594 | 0.414 |
Amazon-Titan-MultiModal | 0.308 | 0.231 | 0.558 | 0.377 |
Jina-V1-CLIP | 0.175 | 0.122 | 0.369 | 0.229 |
Cohere-embedding-v3 | 0.136 | 0.110 | 0.315 | 0.178 |
AmazonProducts-Text2Image Retrieval.
Embedding Model | mAP | R@10 | MRR | nDCG@10 |
---|---|---|---|---|
Marqo-Ecommerce-L | 0.92 | 0.978 | 0.928 | 0.940 |
Marqo-Ecommerce-B | 0.897 | 0.967 | 0.897 | 0.914 |
ViT-SO400M-14-SigLip | 0.860 | 0.954 | 0.860 | 0.882 |
ViT-L-16-SigLip | 0.842 | 0.940 | 0.842 | 0.865 |
GCP-Vertex | 0.808 | 0.933 | 0.808 | 0.837 |
ViT-B-16-SigLip | 0.797 | 0.917 | 0.797 | 0.825 |
Amazon-Titan-MultiModal | 0.762 | 0.889 | 0.763 | 0.791 |
Jina-V1-CLIP | 0.530 | 0.699 | 0.530 | 0.565 |
Cohere-embedding-v3 | 0.433 | 0.597 | 0.433 | 0.465 |
Citation
@software{zhu2024marqoecommembed_2024,
author = {Tianyu Zhu and and Jesse Clark},
month = oct,
title = {{Marqo Ecommerce Embeddings - Foundation Model for Product Embeddings}},
url = {https://github.com/marqo-ai/marqo-ecommerce-embeddings/},
version = {1.0.0},
year = {2024}
}
- Downloads last month
- 484