Datasets:
modelId
stringlengths 4
122
| author
stringlengths 2
42
| last_modified
unknown | downloads
int64 0
392M
| likes
int64 0
6.56k
| library_name
stringclasses 368
values | tags
sequencelengths 1
4.05k
| pipeline_tag
stringclasses 51
values | createdAt
unknown | card
stringlengths 1
1M
|
---|---|---|---|---|---|---|---|---|---|
sentence-transformers/all-mpnet-base-v2 | sentence-transformers | "2024-11-05T15:25:48" | 391,757,489 | 874 | sentence-transformers | [
"sentence-transformers",
"pytorch",
"onnx",
"safetensors",
"openvino",
"mpnet",
"fill-mask",
"feature-extraction",
"sentence-similarity",
"transformers",
"en",
"dataset:s2orc",
"dataset:flax-sentence-embeddings/stackexchange_xml",
"dataset:ms_marco",
"dataset:gooaq",
"dataset:yahoo_answers_topics",
"dataset:code_search_net",
"dataset:search_qa",
"dataset:eli5",
"dataset:snli",
"dataset:multi_nli",
"dataset:wikihow",
"dataset:natural_questions",
"dataset:trivia_qa",
"dataset:embedding-data/sentence-compression",
"dataset:embedding-data/flickr30k-captions",
"dataset:embedding-data/altlex",
"dataset:embedding-data/simple-wiki",
"dataset:embedding-data/QQP",
"dataset:embedding-data/SPECTER",
"dataset:embedding-data/PAQ_pairs",
"dataset:embedding-data/WikiAnswers",
"arxiv:1904.06472",
"arxiv:2102.07033",
"arxiv:2104.08727",
"arxiv:1704.05179",
"arxiv:1810.09305",
"license:apache-2.0",
"autotrain_compatible",
"endpoints_compatible",
"region:us"
] | sentence-similarity | "2022-03-02T23:29:05" | ---
language: en
license: apache-2.0
library_name: sentence-transformers
tags:
- sentence-transformers
- feature-extraction
- sentence-similarity
- transformers
datasets:
- s2orc
- flax-sentence-embeddings/stackexchange_xml
- ms_marco
- gooaq
- yahoo_answers_topics
- code_search_net
- search_qa
- eli5
- snli
- multi_nli
- wikihow
- natural_questions
- trivia_qa
- embedding-data/sentence-compression
- embedding-data/flickr30k-captions
- embedding-data/altlex
- embedding-data/simple-wiki
- embedding-data/QQP
- embedding-data/SPECTER
- embedding-data/PAQ_pairs
- embedding-data/WikiAnswers
pipeline_tag: sentence-similarity
---
# all-mpnet-base-v2
This is a [sentence-transformers](https://www.SBERT.net) model: It maps sentences & paragraphs to a 768 dimensional dense vector space and can be used for tasks like clustering or semantic search.
## Usage (Sentence-Transformers)
Using this model becomes easy when you have [sentence-transformers](https://www.SBERT.net) installed:
```
pip install -U sentence-transformers
```
Then you can use the model like this:
```python
from sentence_transformers import SentenceTransformer
sentences = ["This is an example sentence", "Each sentence is converted"]
model = SentenceTransformer('sentence-transformers/all-mpnet-base-v2')
embeddings = model.encode(sentences)
print(embeddings)
```
## Usage (HuggingFace Transformers)
Without [sentence-transformers](https://www.SBERT.net), you can use the model like this: First, you pass your input through the transformer model, then you have to apply the right pooling-operation on-top of the contextualized word embeddings.
```python
from transformers import AutoTokenizer, AutoModel
import torch
import torch.nn.functional as F
#Mean Pooling - Take attention mask into account for correct averaging
def mean_pooling(model_output, attention_mask):
token_embeddings = model_output[0] #First element of model_output contains all token embeddings
input_mask_expanded = attention_mask.unsqueeze(-1).expand(token_embeddings.size()).float()
return torch.sum(token_embeddings * input_mask_expanded, 1) / torch.clamp(input_mask_expanded.sum(1), min=1e-9)
# Sentences we want sentence embeddings for
sentences = ['This is an example sentence', 'Each sentence is converted']
# Load model from HuggingFace Hub
tokenizer = AutoTokenizer.from_pretrained('sentence-transformers/all-mpnet-base-v2')
model = AutoModel.from_pretrained('sentence-transformers/all-mpnet-base-v2')
# Tokenize sentences
encoded_input = tokenizer(sentences, padding=True, truncation=True, return_tensors='pt')
# Compute token embeddings
with torch.no_grad():
model_output = model(**encoded_input)
# Perform pooling
sentence_embeddings = mean_pooling(model_output, encoded_input['attention_mask'])
# Normalize embeddings
sentence_embeddings = F.normalize(sentence_embeddings, p=2, dim=1)
print("Sentence embeddings:")
print(sentence_embeddings)
```
## Evaluation Results
For an automated evaluation of this model, see the *Sentence Embeddings Benchmark*: [https://seb.sbert.net](https://seb.sbert.net?model_name=sentence-transformers/all-mpnet-base-v2)
------
## Background
The project aims to train sentence embedding models on very large sentence level datasets using a self-supervised
contrastive learning objective. We used the pretrained [`microsoft/mpnet-base`](https://huggingface.co/microsoft/mpnet-base) model and fine-tuned in on a
1B sentence pairs dataset. We use a contrastive learning objective: given a sentence from the pair, the model should predict which out of a set of randomly sampled other sentences, was actually paired with it in our dataset.
We developped this model during the
[Community week using JAX/Flax for NLP & CV](https://discuss.huggingface.co/t/open-to-the-community-community-week-using-jax-flax-for-nlp-cv/7104),
organized by Hugging Face. We developped this model as part of the project:
[Train the Best Sentence Embedding Model Ever with 1B Training Pairs](https://discuss.huggingface.co/t/train-the-best-sentence-embedding-model-ever-with-1b-training-pairs/7354). We benefited from efficient hardware infrastructure to run the project: 7 TPUs v3-8, as well as intervention from Googles Flax, JAX, and Cloud team member about efficient deep learning frameworks.
## Intended uses
Our model is intented to be used as a sentence and short paragraph encoder. Given an input text, it ouptuts a vector which captures
the semantic information. The sentence vector may be used for information retrieval, clustering or sentence similarity tasks.
By default, input text longer than 384 word pieces is truncated.
## Training procedure
### Pre-training
We use the pretrained [`microsoft/mpnet-base`](https://huggingface.co/microsoft/mpnet-base) model. Please refer to the model card for more detailed information about the pre-training procedure.
### Fine-tuning
We fine-tune the model using a contrastive objective. Formally, we compute the cosine similarity from each possible sentence pairs from the batch.
We then apply the cross entropy loss by comparing with true pairs.
#### Hyper parameters
We trained ou model on a TPU v3-8. We train the model during 100k steps using a batch size of 1024 (128 per TPU core).
We use a learning rate warm up of 500. The sequence length was limited to 128 tokens. We used the AdamW optimizer with
a 2e-5 learning rate. The full training script is accessible in this current repository: `train_script.py`.
#### Training data
We use the concatenation from multiple datasets to fine-tune our model. The total number of sentence pairs is above 1 billion sentences.
We sampled each dataset given a weighted probability which configuration is detailed in the `data_config.json` file.
| Dataset | Paper | Number of training tuples |
|--------------------------------------------------------|:----------------------------------------:|:--------------------------:|
| [Reddit comments (2015-2018)](https://github.com/PolyAI-LDN/conversational-datasets/tree/master/reddit) | [paper](https://arxiv.org/abs/1904.06472) | 726,484,430 |
| [S2ORC](https://github.com/allenai/s2orc) Citation pairs (Abstracts) | [paper](https://aclanthology.org/2020.acl-main.447/) | 116,288,806 |
| [WikiAnswers](https://github.com/afader/oqa#wikianswers-corpus) Duplicate question pairs | [paper](https://doi.org/10.1145/2623330.2623677) | 77,427,422 |
| [PAQ](https://github.com/facebookresearch/PAQ) (Question, Answer) pairs | [paper](https://arxiv.org/abs/2102.07033) | 64,371,441 |
| [S2ORC](https://github.com/allenai/s2orc) Citation pairs (Titles) | [paper](https://aclanthology.org/2020.acl-main.447/) | 52,603,982 |
| [S2ORC](https://github.com/allenai/s2orc) (Title, Abstract) | [paper](https://aclanthology.org/2020.acl-main.447/) | 41,769,185 |
| [Stack Exchange](https://huggingface.co/datasets/flax-sentence-embeddings/stackexchange_xml) (Title, Body) pairs | - | 25,316,456 |
| [Stack Exchange](https://huggingface.co/datasets/flax-sentence-embeddings/stackexchange_xml) (Title+Body, Answer) pairs | - | 21,396,559 |
| [Stack Exchange](https://huggingface.co/datasets/flax-sentence-embeddings/stackexchange_xml) (Title, Answer) pairs | - | 21,396,559 |
| [MS MARCO](https://microsoft.github.io/msmarco/) triplets | [paper](https://doi.org/10.1145/3404835.3462804) | 9,144,553 |
| [GOOAQ: Open Question Answering with Diverse Answer Types](https://github.com/allenai/gooaq) | [paper](https://arxiv.org/pdf/2104.08727.pdf) | 3,012,496 |
| [Yahoo Answers](https://www.kaggle.com/soumikrakshit/yahoo-answers-dataset) (Title, Answer) | [paper](https://proceedings.neurips.cc/paper/2015/hash/250cf8b51c773f3f8dc8b4be867a9a02-Abstract.html) | 1,198,260 |
| [Code Search](https://huggingface.co/datasets/code_search_net) | - | 1,151,414 |
| [COCO](https://cocodataset.org/#home) Image captions | [paper](https://link.springer.com/chapter/10.1007%2F978-3-319-10602-1_48) | 828,395|
| [SPECTER](https://github.com/allenai/specter) citation triplets | [paper](https://doi.org/10.18653/v1/2020.acl-main.207) | 684,100 |
| [Yahoo Answers](https://www.kaggle.com/soumikrakshit/yahoo-answers-dataset) (Question, Answer) | [paper](https://proceedings.neurips.cc/paper/2015/hash/250cf8b51c773f3f8dc8b4be867a9a02-Abstract.html) | 681,164 |
| [Yahoo Answers](https://www.kaggle.com/soumikrakshit/yahoo-answers-dataset) (Title, Question) | [paper](https://proceedings.neurips.cc/paper/2015/hash/250cf8b51c773f3f8dc8b4be867a9a02-Abstract.html) | 659,896 |
| [SearchQA](https://huggingface.co/datasets/search_qa) | [paper](https://arxiv.org/abs/1704.05179) | 582,261 |
| [Eli5](https://huggingface.co/datasets/eli5) | [paper](https://doi.org/10.18653/v1/p19-1346) | 325,475 |
| [Flickr 30k](https://shannon.cs.illinois.edu/DenotationGraph/) | [paper](https://transacl.org/ojs/index.php/tacl/article/view/229/33) | 317,695 |
| [Stack Exchange](https://huggingface.co/datasets/flax-sentence-embeddings/stackexchange_xml) Duplicate questions (titles) | | 304,525 |
| AllNLI ([SNLI](https://nlp.stanford.edu/projects/snli/) and [MultiNLI](https://cims.nyu.edu/~sbowman/multinli/) | [paper SNLI](https://doi.org/10.18653/v1/d15-1075), [paper MultiNLI](https://doi.org/10.18653/v1/n18-1101) | 277,230 |
| [Stack Exchange](https://huggingface.co/datasets/flax-sentence-embeddings/stackexchange_xml) Duplicate questions (bodies) | | 250,519 |
| [Stack Exchange](https://huggingface.co/datasets/flax-sentence-embeddings/stackexchange_xml) Duplicate questions (titles+bodies) | | 250,460 |
| [Sentence Compression](https://github.com/google-research-datasets/sentence-compression) | [paper](https://www.aclweb.org/anthology/D13-1155/) | 180,000 |
| [Wikihow](https://github.com/pvl/wikihow_pairs_dataset) | [paper](https://arxiv.org/abs/1810.09305) | 128,542 |
| [Altlex](https://github.com/chridey/altlex/) | [paper](https://aclanthology.org/P16-1135.pdf) | 112,696 |
| [Quora Question Triplets](https://quoradata.quora.com/First-Quora-Dataset-Release-Question-Pairs) | - | 103,663 |
| [Simple Wikipedia](https://cs.pomona.edu/~dkauchak/simplification/) | [paper](https://www.aclweb.org/anthology/P11-2117/) | 102,225 |
| [Natural Questions (NQ)](https://ai.google.com/research/NaturalQuestions) | [paper](https://transacl.org/ojs/index.php/tacl/article/view/1455) | 100,231 |
| [SQuAD2.0](https://rajpurkar.github.io/SQuAD-explorer/) | [paper](https://aclanthology.org/P18-2124.pdf) | 87,599 |
| [TriviaQA](https://huggingface.co/datasets/trivia_qa) | - | 73,346 |
| **Total** | | **1,170,060,424** | |
nesaorg/benchmark_v0 | nesaorg | "2024-08-19T18:24:49" | 98,012,579 | 1 | null | [
"safetensors",
"model_hub_mixin",
"pytorch_model_hub_mixin",
"region:us"
] | null | "2024-08-13T21:07:37" | ---
tags:
- model_hub_mixin
- pytorch_model_hub_mixin
---
This model has been pushed to the Hub using the [PytorchModelHubMixin](https://huggingface.co/docs/huggingface_hub/package_reference/mixins#huggingface_hub.PyTorchModelHubMixin) integration:
- Library: [More Information Needed]
- Docs: [More Information Needed] |
sentence-transformers/all-MiniLM-L6-v2 | sentence-transformers | "2024-11-01T10:26:30" | 74,110,727 | 2,526 | sentence-transformers | [
"sentence-transformers",
"pytorch",
"tf",
"rust",
"onnx",
"safetensors",
"openvino",
"bert",
"feature-extraction",
"sentence-similarity",
"transformers",
"en",
"dataset:s2orc",
"dataset:flax-sentence-embeddings/stackexchange_xml",
"dataset:ms_marco",
"dataset:gooaq",
"dataset:yahoo_answers_topics",
"dataset:code_search_net",
"dataset:search_qa",
"dataset:eli5",
"dataset:snli",
"dataset:multi_nli",
"dataset:wikihow",
"dataset:natural_questions",
"dataset:trivia_qa",
"dataset:embedding-data/sentence-compression",
"dataset:embedding-data/flickr30k-captions",
"dataset:embedding-data/altlex",
"dataset:embedding-data/simple-wiki",
"dataset:embedding-data/QQP",
"dataset:embedding-data/SPECTER",
"dataset:embedding-data/PAQ_pairs",
"dataset:embedding-data/WikiAnswers",
"arxiv:1904.06472",
"arxiv:2102.07033",
"arxiv:2104.08727",
"arxiv:1704.05179",
"arxiv:1810.09305",
"license:apache-2.0",
"autotrain_compatible",
"text-embeddings-inference",
"endpoints_compatible",
"region:us"
] | sentence-similarity | "2022-03-02T23:29:05" | ---
language: en
license: apache-2.0
library_name: sentence-transformers
tags:
- sentence-transformers
- feature-extraction
- sentence-similarity
- transformers
datasets:
- s2orc
- flax-sentence-embeddings/stackexchange_xml
- ms_marco
- gooaq
- yahoo_answers_topics
- code_search_net
- search_qa
- eli5
- snli
- multi_nli
- wikihow
- natural_questions
- trivia_qa
- embedding-data/sentence-compression
- embedding-data/flickr30k-captions
- embedding-data/altlex
- embedding-data/simple-wiki
- embedding-data/QQP
- embedding-data/SPECTER
- embedding-data/PAQ_pairs
- embedding-data/WikiAnswers
pipeline_tag: sentence-similarity
---
# all-MiniLM-L6-v2
This is a [sentence-transformers](https://www.SBERT.net) model: It maps sentences & paragraphs to a 384 dimensional dense vector space and can be used for tasks like clustering or semantic search.
## Usage (Sentence-Transformers)
Using this model becomes easy when you have [sentence-transformers](https://www.SBERT.net) installed:
```
pip install -U sentence-transformers
```
Then you can use the model like this:
```python
from sentence_transformers import SentenceTransformer
sentences = ["This is an example sentence", "Each sentence is converted"]
model = SentenceTransformer('sentence-transformers/all-MiniLM-L6-v2')
embeddings = model.encode(sentences)
print(embeddings)
```
## Usage (HuggingFace Transformers)
Without [sentence-transformers](https://www.SBERT.net), you can use the model like this: First, you pass your input through the transformer model, then you have to apply the right pooling-operation on-top of the contextualized word embeddings.
```python
from transformers import AutoTokenizer, AutoModel
import torch
import torch.nn.functional as F
#Mean Pooling - Take attention mask into account for correct averaging
def mean_pooling(model_output, attention_mask):
token_embeddings = model_output[0] #First element of model_output contains all token embeddings
input_mask_expanded = attention_mask.unsqueeze(-1).expand(token_embeddings.size()).float()
return torch.sum(token_embeddings * input_mask_expanded, 1) / torch.clamp(input_mask_expanded.sum(1), min=1e-9)
# Sentences we want sentence embeddings for
sentences = ['This is an example sentence', 'Each sentence is converted']
# Load model from HuggingFace Hub
tokenizer = AutoTokenizer.from_pretrained('sentence-transformers/all-MiniLM-L6-v2')
model = AutoModel.from_pretrained('sentence-transformers/all-MiniLM-L6-v2')
# Tokenize sentences
encoded_input = tokenizer(sentences, padding=True, truncation=True, return_tensors='pt')
# Compute token embeddings
with torch.no_grad():
model_output = model(**encoded_input)
# Perform pooling
sentence_embeddings = mean_pooling(model_output, encoded_input['attention_mask'])
# Normalize embeddings
sentence_embeddings = F.normalize(sentence_embeddings, p=2, dim=1)
print("Sentence embeddings:")
print(sentence_embeddings)
```
## Evaluation Results
For an automated evaluation of this model, see the *Sentence Embeddings Benchmark*: [https://seb.sbert.net](https://seb.sbert.net?model_name=sentence-transformers/all-MiniLM-L6-v2)
------
## Background
The project aims to train sentence embedding models on very large sentence level datasets using a self-supervised
contrastive learning objective. We used the pretrained [`nreimers/MiniLM-L6-H384-uncased`](https://huggingface.co/nreimers/MiniLM-L6-H384-uncased) model and fine-tuned in on a
1B sentence pairs dataset. We use a contrastive learning objective: given a sentence from the pair, the model should predict which out of a set of randomly sampled other sentences, was actually paired with it in our dataset.
We developed this model during the
[Community week using JAX/Flax for NLP & CV](https://discuss.huggingface.co/t/open-to-the-community-community-week-using-jax-flax-for-nlp-cv/7104),
organized by Hugging Face. We developed this model as part of the project:
[Train the Best Sentence Embedding Model Ever with 1B Training Pairs](https://discuss.huggingface.co/t/train-the-best-sentence-embedding-model-ever-with-1b-training-pairs/7354). We benefited from efficient hardware infrastructure to run the project: 7 TPUs v3-8, as well as intervention from Googles Flax, JAX, and Cloud team member about efficient deep learning frameworks.
## Intended uses
Our model is intended to be used as a sentence and short paragraph encoder. Given an input text, it outputs a vector which captures
the semantic information. The sentence vector may be used for information retrieval, clustering or sentence similarity tasks.
By default, input text longer than 256 word pieces is truncated.
## Training procedure
### Pre-training
We use the pretrained [`nreimers/MiniLM-L6-H384-uncased`](https://huggingface.co/nreimers/MiniLM-L6-H384-uncased) model. Please refer to the model card for more detailed information about the pre-training procedure.
### Fine-tuning
We fine-tune the model using a contrastive objective. Formally, we compute the cosine similarity from each possible sentence pairs from the batch.
We then apply the cross entropy loss by comparing with true pairs.
#### Hyper parameters
We trained our model on a TPU v3-8. We train the model during 100k steps using a batch size of 1024 (128 per TPU core).
We use a learning rate warm up of 500. The sequence length was limited to 128 tokens. We used the AdamW optimizer with
a 2e-5 learning rate. The full training script is accessible in this current repository: `train_script.py`.
#### Training data
We use the concatenation from multiple datasets to fine-tune our model. The total number of sentence pairs is above 1 billion sentences.
We sampled each dataset given a weighted probability which configuration is detailed in the `data_config.json` file.
| Dataset | Paper | Number of training tuples |
|--------------------------------------------------------|:----------------------------------------:|:--------------------------:|
| [Reddit comments (2015-2018)](https://github.com/PolyAI-LDN/conversational-datasets/tree/master/reddit) | [paper](https://arxiv.org/abs/1904.06472) | 726,484,430 |
| [S2ORC](https://github.com/allenai/s2orc) Citation pairs (Abstracts) | [paper](https://aclanthology.org/2020.acl-main.447/) | 116,288,806 |
| [WikiAnswers](https://github.com/afader/oqa#wikianswers-corpus) Duplicate question pairs | [paper](https://doi.org/10.1145/2623330.2623677) | 77,427,422 |
| [PAQ](https://github.com/facebookresearch/PAQ) (Question, Answer) pairs | [paper](https://arxiv.org/abs/2102.07033) | 64,371,441 |
| [S2ORC](https://github.com/allenai/s2orc) Citation pairs (Titles) | [paper](https://aclanthology.org/2020.acl-main.447/) | 52,603,982 |
| [S2ORC](https://github.com/allenai/s2orc) (Title, Abstract) | [paper](https://aclanthology.org/2020.acl-main.447/) | 41,769,185 |
| [Stack Exchange](https://huggingface.co/datasets/flax-sentence-embeddings/stackexchange_xml) (Title, Body) pairs | - | 25,316,456 |
| [Stack Exchange](https://huggingface.co/datasets/flax-sentence-embeddings/stackexchange_xml) (Title+Body, Answer) pairs | - | 21,396,559 |
| [Stack Exchange](https://huggingface.co/datasets/flax-sentence-embeddings/stackexchange_xml) (Title, Answer) pairs | - | 21,396,559 |
| [MS MARCO](https://microsoft.github.io/msmarco/) triplets | [paper](https://doi.org/10.1145/3404835.3462804) | 9,144,553 |
| [GOOAQ: Open Question Answering with Diverse Answer Types](https://github.com/allenai/gooaq) | [paper](https://arxiv.org/pdf/2104.08727.pdf) | 3,012,496 |
| [Yahoo Answers](https://www.kaggle.com/soumikrakshit/yahoo-answers-dataset) (Title, Answer) | [paper](https://proceedings.neurips.cc/paper/2015/hash/250cf8b51c773f3f8dc8b4be867a9a02-Abstract.html) | 1,198,260 |
| [Code Search](https://huggingface.co/datasets/code_search_net) | - | 1,151,414 |
| [COCO](https://cocodataset.org/#home) Image captions | [paper](https://link.springer.com/chapter/10.1007%2F978-3-319-10602-1_48) | 828,395|
| [SPECTER](https://github.com/allenai/specter) citation triplets | [paper](https://doi.org/10.18653/v1/2020.acl-main.207) | 684,100 |
| [Yahoo Answers](https://www.kaggle.com/soumikrakshit/yahoo-answers-dataset) (Question, Answer) | [paper](https://proceedings.neurips.cc/paper/2015/hash/250cf8b51c773f3f8dc8b4be867a9a02-Abstract.html) | 681,164 |
| [Yahoo Answers](https://www.kaggle.com/soumikrakshit/yahoo-answers-dataset) (Title, Question) | [paper](https://proceedings.neurips.cc/paper/2015/hash/250cf8b51c773f3f8dc8b4be867a9a02-Abstract.html) | 659,896 |
| [SearchQA](https://huggingface.co/datasets/search_qa) | [paper](https://arxiv.org/abs/1704.05179) | 582,261 |
| [Eli5](https://huggingface.co/datasets/eli5) | [paper](https://doi.org/10.18653/v1/p19-1346) | 325,475 |
| [Flickr 30k](https://shannon.cs.illinois.edu/DenotationGraph/) | [paper](https://transacl.org/ojs/index.php/tacl/article/view/229/33) | 317,695 |
| [Stack Exchange](https://huggingface.co/datasets/flax-sentence-embeddings/stackexchange_xml) Duplicate questions (titles) | | 304,525 |
| AllNLI ([SNLI](https://nlp.stanford.edu/projects/snli/) and [MultiNLI](https://cims.nyu.edu/~sbowman/multinli/) | [paper SNLI](https://doi.org/10.18653/v1/d15-1075), [paper MultiNLI](https://doi.org/10.18653/v1/n18-1101) | 277,230 |
| [Stack Exchange](https://huggingface.co/datasets/flax-sentence-embeddings/stackexchange_xml) Duplicate questions (bodies) | | 250,519 |
| [Stack Exchange](https://huggingface.co/datasets/flax-sentence-embeddings/stackexchange_xml) Duplicate questions (titles+bodies) | | 250,460 |
| [Sentence Compression](https://github.com/google-research-datasets/sentence-compression) | [paper](https://www.aclweb.org/anthology/D13-1155/) | 180,000 |
| [Wikihow](https://github.com/pvl/wikihow_pairs_dataset) | [paper](https://arxiv.org/abs/1810.09305) | 128,542 |
| [Altlex](https://github.com/chridey/altlex/) | [paper](https://aclanthology.org/P16-1135.pdf) | 112,696 |
| [Quora Question Triplets](https://quoradata.quora.com/First-Quora-Dataset-Release-Question-Pairs) | - | 103,663 |
| [Simple Wikipedia](https://cs.pomona.edu/~dkauchak/simplification/) | [paper](https://www.aclweb.org/anthology/P11-2117/) | 102,225 |
| [Natural Questions (NQ)](https://ai.google.com/research/NaturalQuestions) | [paper](https://transacl.org/ojs/index.php/tacl/article/view/1455) | 100,231 |
| [SQuAD2.0](https://rajpurkar.github.io/SQuAD-explorer/) | [paper](https://aclanthology.org/P18-2124.pdf) | 87,599 |
| [TriviaQA](https://huggingface.co/datasets/trivia_qa) | - | 73,346 |
| **Total** | | **1,170,060,424** | |
google-bert/bert-base-uncased | google-bert | "2024-02-19T11:06:12" | 61,569,952 | 1,897 | transformers | [
"transformers",
"pytorch",
"tf",
"jax",
"rust",
"coreml",
"onnx",
"safetensors",
"bert",
"fill-mask",
"exbert",
"en",
"dataset:bookcorpus",
"dataset:wikipedia",
"arxiv:1810.04805",
"license:apache-2.0",
"autotrain_compatible",
"endpoints_compatible",
"region:us"
] | fill-mask | "2022-03-02T23:29:04" | ---
language: en
tags:
- exbert
license: apache-2.0
datasets:
- bookcorpus
- wikipedia
---
# BERT base model (uncased)
Pretrained model on English language using a masked language modeling (MLM) objective. It was introduced in
[this paper](https://arxiv.org/abs/1810.04805) and first released in
[this repository](https://github.com/google-research/bert). This model is uncased: it does not make a difference
between english and English.
Disclaimer: The team releasing BERT did not write a model card for this model so this model card has been written by
the Hugging Face team.
## Model description
BERT is a transformers model pretrained on a large corpus of English data in a self-supervised fashion. This means it
was pretrained on the raw texts only, with no humans labeling them in any way (which is why it can use lots of
publicly available data) with an automatic process to generate inputs and labels from those texts. More precisely, it
was pretrained with two objectives:
- Masked language modeling (MLM): taking a sentence, the model randomly masks 15% of the words in the input then run
the entire masked sentence through the model and has to predict the masked words. This is different from traditional
recurrent neural networks (RNNs) that usually see the words one after the other, or from autoregressive models like
GPT which internally masks the future tokens. It allows the model to learn a bidirectional representation of the
sentence.
- Next sentence prediction (NSP): the models concatenates two masked sentences as inputs during pretraining. Sometimes
they correspond to sentences that were next to each other in the original text, sometimes not. The model then has to
predict if the two sentences were following each other or not.
This way, the model learns an inner representation of the English language that can then be used to extract features
useful for downstream tasks: if you have a dataset of labeled sentences, for instance, you can train a standard
classifier using the features produced by the BERT model as inputs.
## Model variations
BERT has originally been released in base and large variations, for cased and uncased input text. The uncased models also strips out an accent markers.
Chinese and multilingual uncased and cased versions followed shortly after.
Modified preprocessing with whole word masking has replaced subpiece masking in a following work, with the release of two models.
Other 24 smaller models are released afterward.
The detailed release history can be found on the [google-research/bert readme](https://github.com/google-research/bert/blob/master/README.md) on github.
| Model | #params | Language |
|------------------------|--------------------------------|-------|
| [`bert-base-uncased`](https://huggingface.co/bert-base-uncased) | 110M | English |
| [`bert-large-uncased`](https://huggingface.co/bert-large-uncased) | 340M | English | sub
| [`bert-base-cased`](https://huggingface.co/bert-base-cased) | 110M | English |
| [`bert-large-cased`](https://huggingface.co/bert-large-cased) | 340M | English |
| [`bert-base-chinese`](https://huggingface.co/bert-base-chinese) | 110M | Chinese |
| [`bert-base-multilingual-cased`](https://huggingface.co/bert-base-multilingual-cased) | 110M | Multiple |
| [`bert-large-uncased-whole-word-masking`](https://huggingface.co/bert-large-uncased-whole-word-masking) | 340M | English |
| [`bert-large-cased-whole-word-masking`](https://huggingface.co/bert-large-cased-whole-word-masking) | 340M | English |
## Intended uses & limitations
You can use the raw model for either masked language modeling or next sentence prediction, but it's mostly intended to
be fine-tuned on a downstream task. See the [model hub](https://huggingface.co/models?filter=bert) to look for
fine-tuned versions of a task that interests you.
Note that this model is primarily aimed at being fine-tuned on tasks that use the whole sentence (potentially masked)
to make decisions, such as sequence classification, token classification or question answering. For tasks such as text
generation you should look at model like GPT2.
### How to use
You can use this model directly with a pipeline for masked language modeling:
```python
>>> from transformers import pipeline
>>> unmasker = pipeline('fill-mask', model='bert-base-uncased')
>>> unmasker("Hello I'm a [MASK] model.")
[{'sequence': "[CLS] hello i'm a fashion model. [SEP]",
'score': 0.1073106899857521,
'token': 4827,
'token_str': 'fashion'},
{'sequence': "[CLS] hello i'm a role model. [SEP]",
'score': 0.08774490654468536,
'token': 2535,
'token_str': 'role'},
{'sequence': "[CLS] hello i'm a new model. [SEP]",
'score': 0.05338378623127937,
'token': 2047,
'token_str': 'new'},
{'sequence': "[CLS] hello i'm a super model. [SEP]",
'score': 0.04667217284440994,
'token': 3565,
'token_str': 'super'},
{'sequence': "[CLS] hello i'm a fine model. [SEP]",
'score': 0.027095865458250046,
'token': 2986,
'token_str': 'fine'}]
```
Here is how to use this model to get the features of a given text in PyTorch:
```python
from transformers import BertTokenizer, BertModel
tokenizer = BertTokenizer.from_pretrained('bert-base-uncased')
model = BertModel.from_pretrained("bert-base-uncased")
text = "Replace me by any text you'd like."
encoded_input = tokenizer(text, return_tensors='pt')
output = model(**encoded_input)
```
and in TensorFlow:
```python
from transformers import BertTokenizer, TFBertModel
tokenizer = BertTokenizer.from_pretrained('bert-base-uncased')
model = TFBertModel.from_pretrained("bert-base-uncased")
text = "Replace me by any text you'd like."
encoded_input = tokenizer(text, return_tensors='tf')
output = model(encoded_input)
```
### Limitations and bias
Even if the training data used for this model could be characterized as fairly neutral, this model can have biased
predictions:
```python
>>> from transformers import pipeline
>>> unmasker = pipeline('fill-mask', model='bert-base-uncased')
>>> unmasker("The man worked as a [MASK].")
[{'sequence': '[CLS] the man worked as a carpenter. [SEP]',
'score': 0.09747550636529922,
'token': 10533,
'token_str': 'carpenter'},
{'sequence': '[CLS] the man worked as a waiter. [SEP]',
'score': 0.0523831807076931,
'token': 15610,
'token_str': 'waiter'},
{'sequence': '[CLS] the man worked as a barber. [SEP]',
'score': 0.04962705448269844,
'token': 13362,
'token_str': 'barber'},
{'sequence': '[CLS] the man worked as a mechanic. [SEP]',
'score': 0.03788609802722931,
'token': 15893,
'token_str': 'mechanic'},
{'sequence': '[CLS] the man worked as a salesman. [SEP]',
'score': 0.037680890411138535,
'token': 18968,
'token_str': 'salesman'}]
>>> unmasker("The woman worked as a [MASK].")
[{'sequence': '[CLS] the woman worked as a nurse. [SEP]',
'score': 0.21981462836265564,
'token': 6821,
'token_str': 'nurse'},
{'sequence': '[CLS] the woman worked as a waitress. [SEP]',
'score': 0.1597415804862976,
'token': 13877,
'token_str': 'waitress'},
{'sequence': '[CLS] the woman worked as a maid. [SEP]',
'score': 0.1154729500412941,
'token': 10850,
'token_str': 'maid'},
{'sequence': '[CLS] the woman worked as a prostitute. [SEP]',
'score': 0.037968918681144714,
'token': 19215,
'token_str': 'prostitute'},
{'sequence': '[CLS] the woman worked as a cook. [SEP]',
'score': 0.03042375110089779,
'token': 5660,
'token_str': 'cook'}]
```
This bias will also affect all fine-tuned versions of this model.
## Training data
The BERT model was pretrained on [BookCorpus](https://yknzhu.wixsite.com/mbweb), a dataset consisting of 11,038
unpublished books and [English Wikipedia](https://en.wikipedia.org/wiki/English_Wikipedia) (excluding lists, tables and
headers).
## Training procedure
### Preprocessing
The texts are lowercased and tokenized using WordPiece and a vocabulary size of 30,000. The inputs of the model are
then of the form:
```
[CLS] Sentence A [SEP] Sentence B [SEP]
```
With probability 0.5, sentence A and sentence B correspond to two consecutive sentences in the original corpus, and in
the other cases, it's another random sentence in the corpus. Note that what is considered a sentence here is a
consecutive span of text usually longer than a single sentence. The only constrain is that the result with the two
"sentences" has a combined length of less than 512 tokens.
The details of the masking procedure for each sentence are the following:
- 15% of the tokens are masked.
- In 80% of the cases, the masked tokens are replaced by `[MASK]`.
- In 10% of the cases, the masked tokens are replaced by a random token (different) from the one they replace.
- In the 10% remaining cases, the masked tokens are left as is.
### Pretraining
The model was trained on 4 cloud TPUs in Pod configuration (16 TPU chips total) for one million steps with a batch size
of 256. The sequence length was limited to 128 tokens for 90% of the steps and 512 for the remaining 10%. The optimizer
used is Adam with a learning rate of 1e-4, \\(\beta_{1} = 0.9\\) and \\(\beta_{2} = 0.999\\), a weight decay of 0.01,
learning rate warmup for 10,000 steps and linear decay of the learning rate after.
## Evaluation results
When fine-tuned on downstream tasks, this model achieves the following results:
Glue test results:
| Task | MNLI-(m/mm) | QQP | QNLI | SST-2 | CoLA | STS-B | MRPC | RTE | Average |
|:----:|:-----------:|:----:|:----:|:-----:|:----:|:-----:|:----:|:----:|:-------:|
| | 84.6/83.4 | 71.2 | 90.5 | 93.5 | 52.1 | 85.8 | 88.9 | 66.4 | 79.6 |
### BibTeX entry and citation info
```bibtex
@article{DBLP:journals/corr/abs-1810-04805,
author = {Jacob Devlin and
Ming{-}Wei Chang and
Kenton Lee and
Kristina Toutanova},
title = {{BERT:} Pre-training of Deep Bidirectional Transformers for Language
Understanding},
journal = {CoRR},
volume = {abs/1810.04805},
year = {2018},
url = {http://arxiv.org/abs/1810.04805},
archivePrefix = {arXiv},
eprint = {1810.04805},
timestamp = {Tue, 30 Oct 2018 20:39:56 +0100},
biburl = {https://dblp.org/rec/journals/corr/abs-1810-04805.bib},
bibsource = {dblp computer science bibliography, https://dblp.org}
}
```
<a href="https://huggingface.co/exbert/?model=bert-base-uncased">
<img width="300px" src="https://cdn-media.huggingface.co/exbert/button.png">
</a>
|
FacebookAI/xlm-roberta-large | FacebookAI | "2024-02-19T12:48:30" | 57,186,100 | 345 | transformers | [
"transformers",
"pytorch",
"tf",
"jax",
"onnx",
"safetensors",
"xlm-roberta",
"fill-mask",
"exbert",
"multilingual",
"af",
"am",
"ar",
"as",
"az",
"be",
"bg",
"bn",
"br",
"bs",
"ca",
"cs",
"cy",
"da",
"de",
"el",
"en",
"eo",
"es",
"et",
"eu",
"fa",
"fi",
"fr",
"fy",
"ga",
"gd",
"gl",
"gu",
"ha",
"he",
"hi",
"hr",
"hu",
"hy",
"id",
"is",
"it",
"ja",
"jv",
"ka",
"kk",
"km",
"kn",
"ko",
"ku",
"ky",
"la",
"lo",
"lt",
"lv",
"mg",
"mk",
"ml",
"mn",
"mr",
"ms",
"my",
"ne",
"nl",
"no",
"om",
"or",
"pa",
"pl",
"ps",
"pt",
"ro",
"ru",
"sa",
"sd",
"si",
"sk",
"sl",
"so",
"sq",
"sr",
"su",
"sv",
"sw",
"ta",
"te",
"th",
"tl",
"tr",
"ug",
"uk",
"ur",
"uz",
"vi",
"xh",
"yi",
"zh",
"arxiv:1911.02116",
"license:mit",
"autotrain_compatible",
"endpoints_compatible",
"region:us"
] | fill-mask | "2022-03-02T23:29:04" | ---
tags:
- exbert
language:
- multilingual
- af
- am
- ar
- as
- az
- be
- bg
- bn
- br
- bs
- ca
- cs
- cy
- da
- de
- el
- en
- eo
- es
- et
- eu
- fa
- fi
- fr
- fy
- ga
- gd
- gl
- gu
- ha
- he
- hi
- hr
- hu
- hy
- id
- is
- it
- ja
- jv
- ka
- kk
- km
- kn
- ko
- ku
- ky
- la
- lo
- lt
- lv
- mg
- mk
- ml
- mn
- mr
- ms
- my
- ne
- nl
- no
- om
- or
- pa
- pl
- ps
- pt
- ro
- ru
- sa
- sd
- si
- sk
- sl
- so
- sq
- sr
- su
- sv
- sw
- ta
- te
- th
- tl
- tr
- ug
- uk
- ur
- uz
- vi
- xh
- yi
- zh
license: mit
---
# XLM-RoBERTa (large-sized model)
XLM-RoBERTa model pre-trained on 2.5TB of filtered CommonCrawl data containing 100 languages. It was introduced in the paper [Unsupervised Cross-lingual Representation Learning at Scale](https://arxiv.org/abs/1911.02116) by Conneau et al. and first released in [this repository](https://github.com/pytorch/fairseq/tree/master/examples/xlmr).
Disclaimer: The team releasing XLM-RoBERTa did not write a model card for this model so this model card has been written by the Hugging Face team.
## Model description
XLM-RoBERTa is a multilingual version of RoBERTa. It is pre-trained on 2.5TB of filtered CommonCrawl data containing 100 languages.
RoBERTa is a transformers model pretrained on a large corpus in a self-supervised fashion. This means it was pretrained on the raw texts only, with no humans labelling them in any way (which is why it can use lots of publicly available data) with an automatic process to generate inputs and labels from those texts.
More precisely, it was pretrained with the Masked language modeling (MLM) objective. Taking a sentence, the model randomly masks 15% of the words in the input then run the entire masked sentence through the model and has to predict the masked words. This is different from traditional recurrent neural networks (RNNs) that usually see the words one after the other, or from autoregressive models like GPT which internally mask the future tokens. It allows the model to learn a bidirectional representation of the sentence.
This way, the model learns an inner representation of 100 languages that can then be used to extract features useful for downstream tasks: if you have a dataset of labeled sentences for instance, you can train a standard classifier using the features produced by the XLM-RoBERTa model as inputs.
## Intended uses & limitations
You can use the raw model for masked language modeling, but it's mostly intended to be fine-tuned on a downstream task. See the [model hub](https://huggingface.co/models?search=xlm-roberta) to look for fine-tuned versions on a task that interests you.
Note that this model is primarily aimed at being fine-tuned on tasks that use the whole sentence (potentially masked) to make decisions, such as sequence classification, token classification or question answering. For tasks such as text generation, you should look at models like GPT2.
## Usage
You can use this model directly with a pipeline for masked language modeling:
```python
>>> from transformers import pipeline
>>> unmasker = pipeline('fill-mask', model='xlm-roberta-large')
>>> unmasker("Hello I'm a <mask> model.")
[{'score': 0.10563907772302628,
'sequence': "Hello I'm a fashion model.",
'token': 54543,
'token_str': 'fashion'},
{'score': 0.08015287667512894,
'sequence': "Hello I'm a new model.",
'token': 3525,
'token_str': 'new'},
{'score': 0.033413201570510864,
'sequence': "Hello I'm a model model.",
'token': 3299,
'token_str': 'model'},
{'score': 0.030217764899134636,
'sequence': "Hello I'm a French model.",
'token': 92265,
'token_str': 'French'},
{'score': 0.026436051353812218,
'sequence': "Hello I'm a sexy model.",
'token': 17473,
'token_str': 'sexy'}]
```
Here is how to use this model to get the features of a given text in PyTorch:
```python
from transformers import AutoTokenizer, AutoModelForMaskedLM
tokenizer = AutoTokenizer.from_pretrained('xlm-roberta-large')
model = AutoModelForMaskedLM.from_pretrained("xlm-roberta-large")
# prepare input
text = "Replace me by any text you'd like."
encoded_input = tokenizer(text, return_tensors='pt')
# forward pass
output = model(**encoded_input)
```
### BibTeX entry and citation info
```bibtex
@article{DBLP:journals/corr/abs-1911-02116,
author = {Alexis Conneau and
Kartikay Khandelwal and
Naman Goyal and
Vishrav Chaudhary and
Guillaume Wenzek and
Francisco Guzm{\'{a}}n and
Edouard Grave and
Myle Ott and
Luke Zettlemoyer and
Veselin Stoyanov},
title = {Unsupervised Cross-lingual Representation Learning at Scale},
journal = {CoRR},
volume = {abs/1911.02116},
year = {2019},
url = {http://arxiv.org/abs/1911.02116},
eprinttype = {arXiv},
eprint = {1911.02116},
timestamp = {Mon, 11 Nov 2019 18:38:09 +0100},
biburl = {https://dblp.org/rec/journals/corr/abs-1911-02116.bib},
bibsource = {dblp computer science bibliography, https://dblp.org}
}
```
<a href="https://huggingface.co/exbert/?model=xlm-roberta-base">
<img width="300px" src="https://cdn-media.huggingface.co/exbert/button.png">
</a>
|
openai/whisper-large-v2 | openai | "2024-02-29T10:57:50" | 44,239,856 | 1,649 | transformers | [
"transformers",
"pytorch",
"tf",
"jax",
"safetensors",
"whisper",
"automatic-speech-recognition",
"audio",
"hf-asr-leaderboard",
"en",
"zh",
"de",
"es",
"ru",
"ko",
"fr",
"ja",
"pt",
"tr",
"pl",
"ca",
"nl",
"ar",
"sv",
"it",
"id",
"hi",
"fi",
"vi",
"he",
"uk",
"el",
"ms",
"cs",
"ro",
"da",
"hu",
"ta",
"no",
"th",
"ur",
"hr",
"bg",
"lt",
"la",
"mi",
"ml",
"cy",
"sk",
"te",
"fa",
"lv",
"bn",
"sr",
"az",
"sl",
"kn",
"et",
"mk",
"br",
"eu",
"is",
"hy",
"ne",
"mn",
"bs",
"kk",
"sq",
"sw",
"gl",
"mr",
"pa",
"si",
"km",
"sn",
"yo",
"so",
"af",
"oc",
"ka",
"be",
"tg",
"sd",
"gu",
"am",
"yi",
"lo",
"uz",
"fo",
"ht",
"ps",
"tk",
"nn",
"mt",
"sa",
"lb",
"my",
"bo",
"tl",
"mg",
"as",
"tt",
"haw",
"ln",
"ha",
"ba",
"jw",
"su",
"arxiv:2212.04356",
"license:apache-2.0",
"endpoints_compatible",
"region:us"
] | automatic-speech-recognition | "2022-12-05T18:42:20" | ---
language:
- en
- zh
- de
- es
- ru
- ko
- fr
- ja
- pt
- tr
- pl
- ca
- nl
- ar
- sv
- it
- id
- hi
- fi
- vi
- he
- uk
- el
- ms
- cs
- ro
- da
- hu
- ta
- no
- th
- ur
- hr
- bg
- lt
- la
- mi
- ml
- cy
- sk
- te
- fa
- lv
- bn
- sr
- az
- sl
- kn
- et
- mk
- br
- eu
- is
- hy
- ne
- mn
- bs
- kk
- sq
- sw
- gl
- mr
- pa
- si
- km
- sn
- yo
- so
- af
- oc
- ka
- be
- tg
- sd
- gu
- am
- yi
- lo
- uz
- fo
- ht
- ps
- tk
- nn
- mt
- sa
- lb
- my
- bo
- tl
- mg
- as
- tt
- haw
- ln
- ha
- ba
- jw
- su
tags:
- audio
- automatic-speech-recognition
- hf-asr-leaderboard
widget:
- example_title: Librispeech sample 1
src: https://cdn-media.huggingface.co/speech_samples/sample1.flac
- example_title: Librispeech sample 2
src: https://cdn-media.huggingface.co/speech_samples/sample2.flac
pipeline_tag: automatic-speech-recognition
license: apache-2.0
---
# Whisper
Whisper is a pre-trained model for automatic speech recognition (ASR) and speech translation. Trained on 680k hours
of labelled data, Whisper models demonstrate a strong ability to generalise to many datasets and domains **without** the need
for fine-tuning.
Whisper was proposed in the paper [Robust Speech Recognition via Large-Scale Weak Supervision](https://arxiv.org/abs/2212.04356)
by Alec Radford et al. from OpenAI. The original code repository can be found [here](https://github.com/openai/whisper).
Compared to the Whisper large model, the large-v2 model is trained for 2.5x more epochs with added regularization
for improved performance.
**Disclaimer**: Content for this model card has partly been written by the Hugging Face team, and parts of it were
copied and pasted from the original model card.
## Model details
Whisper is a Transformer based encoder-decoder model, also referred to as a _sequence-to-sequence_ model.
It was trained on 680k hours of labelled speech data annotated using large-scale weak supervision.
The models were trained on either English-only data or multilingual data. The English-only models were trained
on the task of speech recognition. The multilingual models were trained on both speech recognition and speech
translation. For speech recognition, the model predicts transcriptions in the *same* language as the audio.
For speech translation, the model predicts transcriptions to a *different* language to the audio.
Whisper checkpoints come in five configurations of varying model sizes.
The smallest four are trained on either English-only or multilingual data.
The largest checkpoints are multilingual only. All ten of the pre-trained checkpoints
are available on the [Hugging Face Hub](https://huggingface.co/models?search=openai/whisper). The
checkpoints are summarised in the following table with links to the models on the Hub:
| Size | Parameters | English-only | Multilingual |
|----------|------------|------------------------------------------------------|-----------------------------------------------------|
| tiny | 39 M | [✓](https://huggingface.co/openai/whisper-tiny.en) | [✓](https://huggingface.co/openai/whisper-tiny) |
| base | 74 M | [✓](https://huggingface.co/openai/whisper-base.en) | [✓](https://huggingface.co/openai/whisper-base) |
| small | 244 M | [✓](https://huggingface.co/openai/whisper-small.en) | [✓](https://huggingface.co/openai/whisper-small) |
| medium | 769 M | [✓](https://huggingface.co/openai/whisper-medium.en) | [✓](https://huggingface.co/openai/whisper-medium) |
| large | 1550 M | x | [✓](https://huggingface.co/openai/whisper-large) |
| large-v2 | 1550 M | x | [✓](https://huggingface.co/openai/whisper-large-v2) |
# Usage
To transcribe audio samples, the model has to be used alongside a [`WhisperProcessor`](https://huggingface.co/docs/transformers/model_doc/whisper#transformers.WhisperProcessor).
The `WhisperProcessor` is used to:
1. Pre-process the audio inputs (converting them to log-Mel spectrograms for the model)
2. Post-process the model outputs (converting them from tokens to text)
The model is informed of which task to perform (transcription or translation) by passing the appropriate "context tokens". These context tokens
are a sequence of tokens that are given to the decoder at the start of the decoding process, and take the following order:
1. The transcription always starts with the `<|startoftranscript|>` token
2. The second token is the language token (e.g. `<|en|>` for English)
3. The third token is the "task token". It can take one of two values: `<|transcribe|>` for speech recognition or `<|translate|>` for speech translation
4. In addition, a `<|notimestamps|>` token is added if the model should not include timestamp prediction
Thus, a typical sequence of context tokens might look as follows:
```
<|startoftranscript|> <|en|> <|transcribe|> <|notimestamps|>
```
Which tells the model to decode in English, under the task of speech recognition, and not to predict timestamps.
These tokens can either be forced or un-forced. If they are forced, the model is made to predict each token at
each position. This allows one to control the output language and task for the Whisper model. If they are un-forced,
the Whisper model will automatically predict the output langauge and task itself.
The context tokens can be set accordingly:
```python
model.config.forced_decoder_ids = WhisperProcessor.get_decoder_prompt_ids(language="english", task="transcribe")
```
Which forces the model to predict in English under the task of speech recognition.
## Transcription
### English to English
In this example, the context tokens are 'unforced', meaning the model automatically predicts the output language
(English) and task (transcribe).
```python
>>> from transformers import WhisperProcessor, WhisperForConditionalGeneration
>>> from datasets import load_dataset
>>> # load model and processor
>>> processor = WhisperProcessor.from_pretrained("openai/whisper-large-v2")
>>> model = WhisperForConditionalGeneration.from_pretrained("openai/whisper-large-v2")
>>> model.config.forced_decoder_ids = None
>>> # load dummy dataset and read audio files
>>> ds = load_dataset("hf-internal-testing/librispeech_asr_dummy", "clean", split="validation")
>>> sample = ds[0]["audio"]
>>> input_features = processor(sample["array"], sampling_rate=sample["sampling_rate"], return_tensors="pt").input_features
>>> # generate token ids
>>> predicted_ids = model.generate(input_features)
>>> # decode token ids to text
>>> transcription = processor.batch_decode(predicted_ids, skip_special_tokens=False)
['<|startoftranscript|><|en|><|transcribe|><|notimestamps|> Mr. Quilter is the apostle of the middle classes and we are glad to welcome his gospel.<|endoftext|>']
>>> transcription = processor.batch_decode(predicted_ids, skip_special_tokens=True)
[' Mr. Quilter is the apostle of the middle classes and we are glad to welcome his gospel.']
```
The context tokens can be removed from the start of the transcription by setting `skip_special_tokens=True`.
### French to French
The following example demonstrates French to French transcription by setting the decoder ids appropriately.
```python
>>> from transformers import WhisperProcessor, WhisperForConditionalGeneration
>>> from datasets import Audio, load_dataset
>>> # load model and processor
>>> processor = WhisperProcessor.from_pretrained("openai/whisper-large-v2")
>>> model = WhisperForConditionalGeneration.from_pretrained("openai/whisper-large-v2")
>>> forced_decoder_ids = processor.get_decoder_prompt_ids(language="french", task="transcribe")
>>> # load streaming dataset and read first audio sample
>>> ds = load_dataset("common_voice", "fr", split="test", streaming=True)
>>> ds = ds.cast_column("audio", Audio(sampling_rate=16_000))
>>> input_speech = next(iter(ds))["audio"]
>>> input_features = processor(input_speech["array"], sampling_rate=input_speech["sampling_rate"], return_tensors="pt").input_features
>>> # generate token ids
>>> predicted_ids = model.generate(input_features, forced_decoder_ids=forced_decoder_ids)
>>> # decode token ids to text
>>> transcription = processor.batch_decode(predicted_ids)
['<|startoftranscript|><|fr|><|transcribe|><|notimestamps|> Un vrai travail intéressant va enfin être mené sur ce sujet.<|endoftext|>']
>>> transcription = processor.batch_decode(predicted_ids, skip_special_tokens=True)
[' Un vrai travail intéressant va enfin être mené sur ce sujet.']
```
## Translation
Setting the task to "translate" forces the Whisper model to perform speech translation.
### French to English
```python
>>> from transformers import WhisperProcessor, WhisperForConditionalGeneration
>>> from datasets import Audio, load_dataset
>>> # load model and processor
>>> processor = WhisperProcessor.from_pretrained("openai/whisper-large-v2")
>>> model = WhisperForConditionalGeneration.from_pretrained("openai/whisper-large-v2")
>>> forced_decoder_ids = processor.get_decoder_prompt_ids(language="french", task="translate")
>>> # load streaming dataset and read first audio sample
>>> ds = load_dataset("common_voice", "fr", split="test", streaming=True)
>>> ds = ds.cast_column("audio", Audio(sampling_rate=16_000))
>>> input_speech = next(iter(ds))["audio"]
>>> input_features = processor(input_speech["array"], sampling_rate=input_speech["sampling_rate"], return_tensors="pt").input_features
>>> # generate token ids
>>> predicted_ids = model.generate(input_features, forced_decoder_ids=forced_decoder_ids)
>>> # decode token ids to text
>>> transcription = processor.batch_decode(predicted_ids, skip_special_tokens=True)
[' A very interesting work, we will finally be given on this subject.']
```
## Evaluation
This code snippet shows how to evaluate Whisper Large on [LibriSpeech test-clean](https://huggingface.co/datasets/librispeech_asr):
```python
>>> from datasets import load_dataset
>>> from transformers import WhisperForConditionalGeneration, WhisperProcessor
>>> import torch
>>> from evaluate import load
>>> librispeech_test_clean = load_dataset("librispeech_asr", "clean", split="test")
>>> processor = WhisperProcessor.from_pretrained("openai/whisper-large-v2")
>>> model = WhisperForConditionalGeneration.from_pretrained("openai/whisper-large-v2").to("cuda")
>>> def map_to_pred(batch):
>>> audio = batch["audio"]
>>> input_features = processor(audio["array"], sampling_rate=audio["sampling_rate"], return_tensors="pt").input_features
>>> batch["reference"] = processor.tokenizer._normalize(batch['text'])
>>>
>>> with torch.no_grad():
>>> predicted_ids = model.generate(input_features.to("cuda"))[0]
>>> transcription = processor.decode(predicted_ids)
>>> batch["prediction"] = processor.tokenizer._normalize(transcription)
>>> return batch
>>> result = librispeech_test_clean.map(map_to_pred)
>>> wer = load("wer")
>>> print(100 * wer.compute(references=result["reference"], predictions=result["prediction"]))
3.0003583080317572
```
## Long-Form Transcription
The Whisper model is intrinsically designed to work on audio samples of up to 30s in duration. However, by using a chunking
algorithm, it can be used to transcribe audio samples of up to arbitrary length. This is possible through Transformers
[`pipeline`](https://huggingface.co/docs/transformers/main_classes/pipelines#transformers.AutomaticSpeechRecognitionPipeline)
method. Chunking is enabled by setting `chunk_length_s=30` when instantiating the pipeline. With chunking enabled, the pipeline
can be run with batched inference. It can also be extended to predict sequence level timestamps by passing `return_timestamps=True`:
```python
>>> import torch
>>> from transformers import pipeline
>>> from datasets import load_dataset
>>> device = "cuda:0" if torch.cuda.is_available() else "cpu"
>>> pipe = pipeline(
>>> "automatic-speech-recognition",
>>> model="openai/whisper-large-v2",
>>> chunk_length_s=30,
>>> device=device,
>>> )
>>> ds = load_dataset("hf-internal-testing/librispeech_asr_dummy", "clean", split="validation")
>>> sample = ds[0]["audio"]
>>> prediction = pipe(sample.copy(), batch_size=8)["text"]
" Mr. Quilter is the apostle of the middle classes, and we are glad to welcome his gospel."
>>> # we can also return timestamps for the predictions
>>> prediction = pipe(sample.copy(), batch_size=8, return_timestamps=True)["chunks"]
[{'text': ' Mr. Quilter is the apostle of the middle classes and we are glad to welcome his gospel.',
'timestamp': (0.0, 5.44)}]
```
Refer to the blog post [ASR Chunking](https://huggingface.co/blog/asr-chunking) for more details on the chunking algorithm.
## Fine-Tuning
The pre-trained Whisper model demonstrates a strong ability to generalise to different datasets and domains. However,
its predictive capabilities can be improved further for certain languages and tasks through *fine-tuning*. The blog
post [Fine-Tune Whisper with 🤗 Transformers](https://huggingface.co/blog/fine-tune-whisper) provides a step-by-step
guide to fine-tuning the Whisper model with as little as 5 hours of labelled data.
### Evaluated Use
The primary intended users of these models are AI researchers studying robustness, generalization, capabilities, biases, and constraints of the current model. However, Whisper is also potentially quite useful as an ASR solution for developers, especially for English speech recognition. We recognize that once models are released, it is impossible to restrict access to only “intended” uses or to draw reasonable guidelines around what is or is not research.
The models are primarily trained and evaluated on ASR and speech translation to English tasks. They show strong ASR results in ~10 languages. They may exhibit additional capabilities, particularly if fine-tuned on certain tasks like voice activity detection, speaker classification, or speaker diarization but have not been robustly evaluated in these areas. We strongly recommend that users perform robust evaluations of the models in a particular context and domain before deploying them.
In particular, we caution against using Whisper models to transcribe recordings of individuals taken without their consent or purporting to use these models for any kind of subjective classification. We recommend against use in high-risk domains like decision-making contexts, where flaws in accuracy can lead to pronounced flaws in outcomes. The models are intended to transcribe and translate speech, use of the model for classification is not only not evaluated but also not appropriate, particularly to infer human attributes.
## Training Data
The models are trained on 680,000 hours of audio and the corresponding transcripts collected from the internet. 65% of this data (or 438,000 hours) represents English-language audio and matched English transcripts, roughly 18% (or 126,000 hours) represents non-English audio and English transcripts, while the final 17% (or 117,000 hours) represents non-English audio and the corresponding transcript. This non-English data represents 98 different languages.
As discussed in [the accompanying paper](https://cdn.openai.com/papers/whisper.pdf), we see that performance on transcription in a given language is directly correlated with the amount of training data we employ in that language.
## Performance and Limitations
Our studies show that, over many existing ASR systems, the models exhibit improved robustness to accents, background noise, technical language, as well as zero shot translation from multiple languages into English; and that accuracy on speech recognition and translation is near the state-of-the-art level.
However, because the models are trained in a weakly supervised manner using large-scale noisy data, the predictions may include texts that are not actually spoken in the audio input (i.e. hallucination). We hypothesize that this happens because, given their general knowledge of language, the models combine trying to predict the next word in audio with trying to transcribe the audio itself.
Our models perform unevenly across languages, and we observe lower accuracy on low-resource and/or low-discoverability languages or languages where we have less training data. The models also exhibit disparate performance on different accents and dialects of particular languages, which may include higher word error rate across speakers of different genders, races, ages, or other demographic criteria. Our full evaluation results are presented in [the paper accompanying this release](https://cdn.openai.com/papers/whisper.pdf).
In addition, the sequence-to-sequence architecture of the model makes it prone to generating repetitive texts, which can be mitigated to some degree by beam search and temperature scheduling but not perfectly. Further analysis on these limitations are provided in [the paper](https://cdn.openai.com/papers/whisper.pdf). It is likely that this behavior and hallucinations may be worse on lower-resource and/or lower-discoverability languages.
## Broader Implications
We anticipate that Whisper models’ transcription capabilities may be used for improving accessibility tools. While Whisper models cannot be used for real-time transcription out of the box – their speed and size suggest that others may be able to build applications on top of them that allow for near-real-time speech recognition and translation. The real value of beneficial applications built on top of Whisper models suggests that the disparate performance of these models may have real economic implications.
There are also potential dual use concerns that come with releasing Whisper. While we hope the technology will be used primarily for beneficial purposes, making ASR technology more accessible could enable more actors to build capable surveillance technologies or scale up existing surveillance efforts, as the speed and accuracy allow for affordable automatic transcription and translation of large volumes of audio communication. Moreover, these models may have some capabilities to recognize specific individuals out of the box, which in turn presents safety concerns related both to dual use and disparate performance. In practice, we expect that the cost of transcription is not the limiting factor of scaling up surveillance projects.
### BibTeX entry and citation info
```bibtex
@misc{radford2022whisper,
doi = {10.48550/ARXIV.2212.04356},
url = {https://arxiv.org/abs/2212.04356},
author = {Radford, Alec and Kim, Jong Wook and Xu, Tao and Brockman, Greg and McLeavey, Christine and Sutskever, Ilya},
title = {Robust Speech Recognition via Large-Scale Weak Supervision},
publisher = {arXiv},
year = {2022},
copyright = {arXiv.org perpetual, non-exclusive license}
}
```
|
nesaorg/fc_8 | nesaorg | "2024-08-14T12:56:48" | 28,123,640 | 0 | null | [
"safetensors",
"model_hub_mixin",
"pytorch_model_hub_mixin",
"region:us"
] | null | "2024-08-14T12:56:30" | ---
tags:
- model_hub_mixin
- pytorch_model_hub_mixin
---
This model has been pushed to the Hub using the [PytorchModelHubMixin](https://huggingface.co/docs/huggingface_hub/package_reference/mixins#huggingface_hub.PyTorchModelHubMixin) integration:
- Library: [More Information Needed]
- Docs: [More Information Needed] |
openai/clip-vit-base-patch32 | openai | "2024-02-29T09:45:55" | 26,745,969 | 534 | transformers | [
"transformers",
"pytorch",
"tf",
"jax",
"clip",
"zero-shot-image-classification",
"vision",
"arxiv:2103.00020",
"arxiv:1908.04913",
"endpoints_compatible",
"region:us"
] | zero-shot-image-classification | "2022-03-02T23:29:05" | ---
tags:
- vision
widget:
- src: https://huggingface.co/datasets/mishig/sample_images/resolve/main/cat-dog-music.png
candidate_labels: playing music, playing sports
example_title: Cat & Dog
---
# Model Card: CLIP
Disclaimer: The model card is taken and modified from the official CLIP repository, it can be found [here](https://github.com/openai/CLIP/blob/main/model-card.md).
## Model Details
The CLIP model was developed by researchers at OpenAI to learn about what contributes to robustness in computer vision tasks. The model was also developed to test the ability of models to generalize to arbitrary image classification tasks in a zero-shot manner. It was not developed for general model deployment - to deploy models like CLIP, researchers will first need to carefully study their capabilities in relation to the specific context they’re being deployed within.
### Model Date
January 2021
### Model Type
The model uses a ViT-B/32 Transformer architecture as an image encoder and uses a masked self-attention Transformer as a text encoder. These encoders are trained to maximize the similarity of (image, text) pairs via a contrastive loss.
The original implementation had two variants: one using a ResNet image encoder and the other using a Vision Transformer. This repository has the variant with the Vision Transformer.
### Documents
- [Blog Post](https://openai.com/blog/clip/)
- [CLIP Paper](https://arxiv.org/abs/2103.00020)
### Use with Transformers
```python3
from PIL import Image
import requests
from transformers import CLIPProcessor, CLIPModel
model = CLIPModel.from_pretrained("openai/clip-vit-base-patch32")
processor = CLIPProcessor.from_pretrained("openai/clip-vit-base-patch32")
url = "http://images.cocodataset.org/val2017/000000039769.jpg"
image = Image.open(requests.get(url, stream=True).raw)
inputs = processor(text=["a photo of a cat", "a photo of a dog"], images=image, return_tensors="pt", padding=True)
outputs = model(**inputs)
logits_per_image = outputs.logits_per_image # this is the image-text similarity score
probs = logits_per_image.softmax(dim=1) # we can take the softmax to get the label probabilities
```
## Model Use
### Intended Use
The model is intended as a research output for research communities. We hope that this model will enable researchers to better understand and explore zero-shot, arbitrary image classification. We also hope it can be used for interdisciplinary studies of the potential impact of such models - the CLIP paper includes a discussion of potential downstream impacts to provide an example for this sort of analysis.
#### Primary intended uses
The primary intended users of these models are AI researchers.
We primarily imagine the model will be used by researchers to better understand robustness, generalization, and other capabilities, biases, and constraints of computer vision models.
### Out-of-Scope Use Cases
**Any** deployed use case of the model - whether commercial or not - is currently out of scope. Non-deployed use cases such as image search in a constrained environment, are also not recommended unless there is thorough in-domain testing of the model with a specific, fixed class taxonomy. This is because our safety assessment demonstrated a high need for task specific testing especially given the variability of CLIP’s performance with different class taxonomies. This makes untested and unconstrained deployment of the model in any use case currently potentially harmful.
Certain use cases which would fall under the domain of surveillance and facial recognition are always out-of-scope regardless of performance of the model. This is because the use of artificial intelligence for tasks such as these can be premature currently given the lack of testing norms and checks to ensure its fair use.
Since the model has not been purposefully trained in or evaluated on any languages other than English, its use should be limited to English language use cases.
## Data
The model was trained on publicly available image-caption data. This was done through a combination of crawling a handful of websites and using commonly-used pre-existing image datasets such as [YFCC100M](http://projects.dfki.uni-kl.de/yfcc100m/). A large portion of the data comes from our crawling of the internet. This means that the data is more representative of people and societies most connected to the internet which tend to skew towards more developed nations, and younger, male users.
### Data Mission Statement
Our goal with building this dataset was to test out robustness and generalizability in computer vision tasks. As a result, the focus was on gathering large quantities of data from different publicly-available internet data sources. The data was gathered in a mostly non-interventionist manner. However, we only crawled websites that had policies against excessively violent and adult images and allowed us to filter out such content. We do not intend for this dataset to be used as the basis for any commercial or deployed model and will not be releasing the dataset.
## Performance and Limitations
### Performance
We have evaluated the performance of CLIP on a wide range of benchmarks across a variety of computer vision datasets such as OCR to texture recognition to fine-grained classification. The paper describes model performance on the following datasets:
- Food101
- CIFAR10
- CIFAR100
- Birdsnap
- SUN397
- Stanford Cars
- FGVC Aircraft
- VOC2007
- DTD
- Oxford-IIIT Pet dataset
- Caltech101
- Flowers102
- MNIST
- SVHN
- IIIT5K
- Hateful Memes
- SST-2
- UCF101
- Kinetics700
- Country211
- CLEVR Counting
- KITTI Distance
- STL-10
- RareAct
- Flickr30
- MSCOCO
- ImageNet
- ImageNet-A
- ImageNet-R
- ImageNet Sketch
- ObjectNet (ImageNet Overlap)
- Youtube-BB
- ImageNet-Vid
## Limitations
CLIP and our analysis of it have a number of limitations. CLIP currently struggles with respect to certain tasks such as fine grained classification and counting objects. CLIP also poses issues with regards to fairness and bias which we discuss in the paper and briefly in the next section. Additionally, our approach to testing CLIP also has an important limitation- in many cases we have used linear probes to evaluate the performance of CLIP and there is evidence suggesting that linear probes can underestimate model performance.
### Bias and Fairness
We find that the performance of CLIP - and the specific biases it exhibits - can depend significantly on class design and the choices one makes for categories to include and exclude. We tested the risk of certain kinds of denigration with CLIP by classifying images of people from [Fairface](https://arxiv.org/abs/1908.04913) into crime-related and non-human animal categories. We found significant disparities with respect to race and gender. Additionally, we found that these disparities could shift based on how the classes were constructed. (Details captured in the Broader Impacts Section in the paper).
We also tested the performance of CLIP on gender, race and age classification using the Fairface dataset (We default to using race categories as they are constructed in the Fairface dataset.) in order to assess quality of performance across different demographics. We found accuracy >96% across all races for gender classification with ‘Middle Eastern’ having the highest accuracy (98.4%) and ‘White’ having the lowest (96.5%). Additionally, CLIP averaged ~93% for racial classification and ~63% for age classification. Our use of evaluations to test for gender, race and age classification as well as denigration harms is simply to evaluate performance of the model across people and surface potential risks and not to demonstrate an endorsement/enthusiasm for such tasks.
## Feedback
### Where to send questions or comments about the model
Please use [this Google Form](https://forms.gle/Uv7afRH5dvY34ZEs9) |
openai/clip-vit-large-patch14 | openai | "2023-09-15T15:49:35" | 23,609,966 | 1,442 | transformers | [
"transformers",
"pytorch",
"tf",
"jax",
"safetensors",
"clip",
"zero-shot-image-classification",
"vision",
"arxiv:2103.00020",
"arxiv:1908.04913",
"endpoints_compatible",
"region:us"
] | zero-shot-image-classification | "2022-03-02T23:29:05" | ---
tags:
- vision
widget:
- src: https://huggingface.co/datasets/mishig/sample_images/resolve/main/cat-dog-music.png
candidate_labels: playing music, playing sports
example_title: Cat & Dog
---
# Model Card: CLIP
Disclaimer: The model card is taken and modified from the official CLIP repository, it can be found [here](https://github.com/openai/CLIP/blob/main/model-card.md).
## Model Details
The CLIP model was developed by researchers at OpenAI to learn about what contributes to robustness in computer vision tasks. The model was also developed to test the ability of models to generalize to arbitrary image classification tasks in a zero-shot manner. It was not developed for general model deployment - to deploy models like CLIP, researchers will first need to carefully study their capabilities in relation to the specific context they’re being deployed within.
### Model Date
January 2021
### Model Type
The base model uses a ViT-L/14 Transformer architecture as an image encoder and uses a masked self-attention Transformer as a text encoder. These encoders are trained to maximize the similarity of (image, text) pairs via a contrastive loss.
The original implementation had two variants: one using a ResNet image encoder and the other using a Vision Transformer. This repository has the variant with the Vision Transformer.
### Documents
- [Blog Post](https://openai.com/blog/clip/)
- [CLIP Paper](https://arxiv.org/abs/2103.00020)
### Use with Transformers
```python
from PIL import Image
import requests
from transformers import CLIPProcessor, CLIPModel
model = CLIPModel.from_pretrained("openai/clip-vit-large-patch14")
processor = CLIPProcessor.from_pretrained("openai/clip-vit-large-patch14")
url = "http://images.cocodataset.org/val2017/000000039769.jpg"
image = Image.open(requests.get(url, stream=True).raw)
inputs = processor(text=["a photo of a cat", "a photo of a dog"], images=image, return_tensors="pt", padding=True)
outputs = model(**inputs)
logits_per_image = outputs.logits_per_image # this is the image-text similarity score
probs = logits_per_image.softmax(dim=1) # we can take the softmax to get the label probabilities
```
## Model Use
### Intended Use
The model is intended as a research output for research communities. We hope that this model will enable researchers to better understand and explore zero-shot, arbitrary image classification. We also hope it can be used for interdisciplinary studies of the potential impact of such models - the CLIP paper includes a discussion of potential downstream impacts to provide an example for this sort of analysis.
#### Primary intended uses
The primary intended users of these models are AI researchers.
We primarily imagine the model will be used by researchers to better understand robustness, generalization, and other capabilities, biases, and constraints of computer vision models.
### Out-of-Scope Use Cases
**Any** deployed use case of the model - whether commercial or not - is currently out of scope. Non-deployed use cases such as image search in a constrained environment, are also not recommended unless there is thorough in-domain testing of the model with a specific, fixed class taxonomy. This is because our safety assessment demonstrated a high need for task specific testing especially given the variability of CLIP’s performance with different class taxonomies. This makes untested and unconstrained deployment of the model in any use case currently potentially harmful.
Certain use cases which would fall under the domain of surveillance and facial recognition are always out-of-scope regardless of performance of the model. This is because the use of artificial intelligence for tasks such as these can be premature currently given the lack of testing norms and checks to ensure its fair use.
Since the model has not been purposefully trained in or evaluated on any languages other than English, its use should be limited to English language use cases.
## Data
The model was trained on publicly available image-caption data. This was done through a combination of crawling a handful of websites and using commonly-used pre-existing image datasets such as [YFCC100M](http://projects.dfki.uni-kl.de/yfcc100m/). A large portion of the data comes from our crawling of the internet. This means that the data is more representative of people and societies most connected to the internet which tend to skew towards more developed nations, and younger, male users.
### Data Mission Statement
Our goal with building this dataset was to test out robustness and generalizability in computer vision tasks. As a result, the focus was on gathering large quantities of data from different publicly-available internet data sources. The data was gathered in a mostly non-interventionist manner. However, we only crawled websites that had policies against excessively violent and adult images and allowed us to filter out such content. We do not intend for this dataset to be used as the basis for any commercial or deployed model and will not be releasing the dataset.
## Performance and Limitations
### Performance
We have evaluated the performance of CLIP on a wide range of benchmarks across a variety of computer vision datasets such as OCR to texture recognition to fine-grained classification. The paper describes model performance on the following datasets:
- Food101
- CIFAR10
- CIFAR100
- Birdsnap
- SUN397
- Stanford Cars
- FGVC Aircraft
- VOC2007
- DTD
- Oxford-IIIT Pet dataset
- Caltech101
- Flowers102
- MNIST
- SVHN
- IIIT5K
- Hateful Memes
- SST-2
- UCF101
- Kinetics700
- Country211
- CLEVR Counting
- KITTI Distance
- STL-10
- RareAct
- Flickr30
- MSCOCO
- ImageNet
- ImageNet-A
- ImageNet-R
- ImageNet Sketch
- ObjectNet (ImageNet Overlap)
- Youtube-BB
- ImageNet-Vid
## Limitations
CLIP and our analysis of it have a number of limitations. CLIP currently struggles with respect to certain tasks such as fine grained classification and counting objects. CLIP also poses issues with regards to fairness and bias which we discuss in the paper and briefly in the next section. Additionally, our approach to testing CLIP also has an important limitation- in many cases we have used linear probes to evaluate the performance of CLIP and there is evidence suggesting that linear probes can underestimate model performance.
### Bias and Fairness
We find that the performance of CLIP - and the specific biases it exhibits - can depend significantly on class design and the choices one makes for categories to include and exclude. We tested the risk of certain kinds of denigration with CLIP by classifying images of people from [Fairface](https://arxiv.org/abs/1908.04913) into crime-related and non-human animal categories. We found significant disparities with respect to race and gender. Additionally, we found that these disparities could shift based on how the classes were constructed. (Details captured in the Broader Impacts Section in the paper).
We also tested the performance of CLIP on gender, race and age classification using the Fairface dataset (We default to using race categories as they are constructed in the Fairface dataset.) in order to assess quality of performance across different demographics. We found accuracy >96% across all races for gender classification with ‘Middle Eastern’ having the highest accuracy (98.4%) and ‘White’ having the lowest (96.5%). Additionally, CLIP averaged ~93% for racial classification and ~63% for age classification. Our use of evaluations to test for gender, race and age classification as well as denigration harms is simply to evaluate performance of the model across people and surface potential risks and not to demonstrate an endorsement/enthusiasm for such tasks.
## Feedback
### Where to send questions or comments about the model
Please use [this Google Form](https://forms.gle/Uv7afRH5dvY34ZEs9) |
jonatasgrosman/wav2vec2-large-xlsr-53-english | jonatasgrosman | "2023-03-25T10:56:55" | 23,527,016 | 445 | transformers | [
"transformers",
"pytorch",
"jax",
"safetensors",
"wav2vec2",
"automatic-speech-recognition",
"audio",
"en",
"hf-asr-leaderboard",
"mozilla-foundation/common_voice_6_0",
"robust-speech-event",
"speech",
"xlsr-fine-tuning-week",
"dataset:common_voice",
"dataset:mozilla-foundation/common_voice_6_0",
"license:apache-2.0",
"model-index",
"endpoints_compatible",
"region:us"
] | automatic-speech-recognition | "2022-03-02T23:29:05" | ---
language: en
datasets:
- common_voice
- mozilla-foundation/common_voice_6_0
metrics:
- wer
- cer
tags:
- audio
- automatic-speech-recognition
- en
- hf-asr-leaderboard
- mozilla-foundation/common_voice_6_0
- robust-speech-event
- speech
- xlsr-fine-tuning-week
license: apache-2.0
model-index:
- name: XLSR Wav2Vec2 English by Jonatas Grosman
results:
- task:
name: Automatic Speech Recognition
type: automatic-speech-recognition
dataset:
name: Common Voice en
type: common_voice
args: en
metrics:
- name: Test WER
type: wer
value: 19.06
- name: Test CER
type: cer
value: 7.69
- name: Test WER (+LM)
type: wer
value: 14.81
- name: Test CER (+LM)
type: cer
value: 6.84
- task:
name: Automatic Speech Recognition
type: automatic-speech-recognition
dataset:
name: Robust Speech Event - Dev Data
type: speech-recognition-community-v2/dev_data
args: en
metrics:
- name: Dev WER
type: wer
value: 27.72
- name: Dev CER
type: cer
value: 11.65
- name: Dev WER (+LM)
type: wer
value: 20.85
- name: Dev CER (+LM)
type: cer
value: 11.01
---
# Fine-tuned XLSR-53 large model for speech recognition in English
Fine-tuned [facebook/wav2vec2-large-xlsr-53](https://huggingface.co/facebook/wav2vec2-large-xlsr-53) on English using the train and validation splits of [Common Voice 6.1](https://huggingface.co/datasets/common_voice).
When using this model, make sure that your speech input is sampled at 16kHz.
This model has been fine-tuned thanks to the GPU credits generously given by the [OVHcloud](https://www.ovhcloud.com/en/public-cloud/ai-training/) :)
The script used for training can be found here: https://github.com/jonatasgrosman/wav2vec2-sprint
## Usage
The model can be used directly (without a language model) as follows...
Using the [HuggingSound](https://github.com/jonatasgrosman/huggingsound) library:
```python
from huggingsound import SpeechRecognitionModel
model = SpeechRecognitionModel("jonatasgrosman/wav2vec2-large-xlsr-53-english")
audio_paths = ["/path/to/file.mp3", "/path/to/another_file.wav"]
transcriptions = model.transcribe(audio_paths)
```
Writing your own inference script:
```python
import torch
import librosa
from datasets import load_dataset
from transformers import Wav2Vec2ForCTC, Wav2Vec2Processor
LANG_ID = "en"
MODEL_ID = "jonatasgrosman/wav2vec2-large-xlsr-53-english"
SAMPLES = 10
test_dataset = load_dataset("common_voice", LANG_ID, split=f"test[:{SAMPLES}]")
processor = Wav2Vec2Processor.from_pretrained(MODEL_ID)
model = Wav2Vec2ForCTC.from_pretrained(MODEL_ID)
# Preprocessing the datasets.
# We need to read the audio files as arrays
def speech_file_to_array_fn(batch):
speech_array, sampling_rate = librosa.load(batch["path"], sr=16_000)
batch["speech"] = speech_array
batch["sentence"] = batch["sentence"].upper()
return batch
test_dataset = test_dataset.map(speech_file_to_array_fn)
inputs = processor(test_dataset["speech"], sampling_rate=16_000, return_tensors="pt", padding=True)
with torch.no_grad():
logits = model(inputs.input_values, attention_mask=inputs.attention_mask).logits
predicted_ids = torch.argmax(logits, dim=-1)
predicted_sentences = processor.batch_decode(predicted_ids)
for i, predicted_sentence in enumerate(predicted_sentences):
print("-" * 100)
print("Reference:", test_dataset[i]["sentence"])
print("Prediction:", predicted_sentence)
```
| Reference | Prediction |
| ------------- | ------------- |
| "SHE'LL BE ALL RIGHT." | SHE'LL BE ALL RIGHT |
| SIX | SIX |
| "ALL'S WELL THAT ENDS WELL." | ALL AS WELL THAT ENDS WELL |
| DO YOU MEAN IT? | DO YOU MEAN IT |
| THE NEW PATCH IS LESS INVASIVE THAN THE OLD ONE, BUT STILL CAUSES REGRESSIONS. | THE NEW PATCH IS LESS INVASIVE THAN THE OLD ONE BUT STILL CAUSES REGRESSION |
| HOW IS MOZILLA GOING TO HANDLE AMBIGUITIES LIKE QUEUE AND CUE? | HOW IS MOSLILLAR GOING TO HANDLE ANDBEWOOTH HIS LIKE Q AND Q |
| "I GUESS YOU MUST THINK I'M KINDA BATTY." | RUSTIAN WASTIN PAN ONTE BATTLY |
| NO ONE NEAR THE REMOTE MACHINE YOU COULD RING? | NO ONE NEAR THE REMOTE MACHINE YOU COULD RING |
| SAUCE FOR THE GOOSE IS SAUCE FOR THE GANDER. | SAUCE FOR THE GUICE IS SAUCE FOR THE GONDER |
| GROVES STARTED WRITING SONGS WHEN SHE WAS FOUR YEARS OLD. | GRAFS STARTED WRITING SONGS WHEN SHE WAS FOUR YEARS OLD |
## Evaluation
1. To evaluate on `mozilla-foundation/common_voice_6_0` with split `test`
```bash
python eval.py --model_id jonatasgrosman/wav2vec2-large-xlsr-53-english --dataset mozilla-foundation/common_voice_6_0 --config en --split test
```
2. To evaluate on `speech-recognition-community-v2/dev_data`
```bash
python eval.py --model_id jonatasgrosman/wav2vec2-large-xlsr-53-english --dataset speech-recognition-community-v2/dev_data --config en --split validation --chunk_length_s 5.0 --stride_length_s 1.0
```
## Citation
If you want to cite this model you can use this:
```bibtex
@misc{grosman2021xlsr53-large-english,
title={Fine-tuned {XLSR}-53 large model for speech recognition in {E}nglish},
author={Grosman, Jonatas},
howpublished={\url{https://huggingface.co/jonatasgrosman/wav2vec2-large-xlsr-53-english}},
year={2021}
}
``` |
openai/clip-vit-base-patch16 | openai | "2022-10-04T09:42:28" | 22,726,589 | 97 | transformers | [
"transformers",
"pytorch",
"jax",
"clip",
"zero-shot-image-classification",
"vision",
"arxiv:2103.00020",
"arxiv:1908.04913",
"endpoints_compatible",
"region:us"
] | zero-shot-image-classification | "2022-03-02T23:29:05" | ---
tags:
- vision
widget:
- src: https://huggingface.co/datasets/mishig/sample_images/resolve/main/cat-dog-music.png
candidate_labels: playing music, playing sports
example_title: Cat & Dog
---
# Model Card: CLIP
Disclaimer: The model card is taken and modified from the official CLIP repository, it can be found [here](https://github.com/openai/CLIP/blob/main/model-card.md).
## Model Details
The CLIP model was developed by researchers at OpenAI to learn about what contributes to robustness in computer vision tasks. The model was also developed to test the ability of models to generalize to arbitrary image classification tasks in a zero-shot manner. It was not developed for general model deployment - to deploy models like CLIP, researchers will first need to carefully study their capabilities in relation to the specific context they’re being deployed within.
### Model Date
January 2021
### Model Type
The base model uses a ViT-B/16 Transformer architecture as an image encoder and uses a masked self-attention Transformer as a text encoder. These encoders are trained to maximize the similarity of (image, text) pairs via a contrastive loss.
The original implementation had two variants: one using a ResNet image encoder and the other using a Vision Transformer. This repository has the variant with the Vision Transformer.
### Documents
- [Blog Post](https://openai.com/blog/clip/)
- [CLIP Paper](https://arxiv.org/abs/2103.00020)
### Use with Transformers
```python3
from PIL import Image
import requests
from transformers import CLIPProcessor, CLIPModel
model = CLIPModel.from_pretrained("openai/clip-vit-base-patch16")
processor = CLIPProcessor.from_pretrained("openai/clip-vit-base-patch16")
url = "http://images.cocodataset.org/val2017/000000039769.jpg"
image = Image.open(requests.get(url, stream=True).raw)
inputs = processor(text=["a photo of a cat", "a photo of a dog"], images=image, return_tensors="pt", padding=True)
outputs = model(**inputs)
logits_per_image = outputs.logits_per_image # this is the image-text similarity score
probs = logits_per_image.softmax(dim=1) # we can take the softmax to get the label probabilities
```
## Model Use
### Intended Use
The model is intended as a research output for research communities. We hope that this model will enable researchers to better understand and explore zero-shot, arbitrary image classification. We also hope it can be used for interdisciplinary studies of the potential impact of such models - the CLIP paper includes a discussion of potential downstream impacts to provide an example for this sort of analysis.
#### Primary intended uses
The primary intended users of these models are AI researchers.
We primarily imagine the model will be used by researchers to better understand robustness, generalization, and other capabilities, biases, and constraints of computer vision models.
### Out-of-Scope Use Cases
**Any** deployed use case of the model - whether commercial or not - is currently out of scope. Non-deployed use cases such as image search in a constrained environment, are also not recommended unless there is thorough in-domain testing of the model with a specific, fixed class taxonomy. This is because our safety assessment demonstrated a high need for task specific testing especially given the variability of CLIP’s performance with different class taxonomies. This makes untested and unconstrained deployment of the model in any use case currently potentially harmful.
Certain use cases which would fall under the domain of surveillance and facial recognition are always out-of-scope regardless of performance of the model. This is because the use of artificial intelligence for tasks such as these can be premature currently given the lack of testing norms and checks to ensure its fair use.
Since the model has not been purposefully trained in or evaluated on any languages other than English, its use should be limited to English language use cases.
## Data
The model was trained on publicly available image-caption data. This was done through a combination of crawling a handful of websites and using commonly-used pre-existing image datasets such as [YFCC100M](http://projects.dfki.uni-kl.de/yfcc100m/). A large portion of the data comes from our crawling of the internet. This means that the data is more representative of people and societies most connected to the internet which tend to skew towards more developed nations, and younger, male users.
### Data Mission Statement
Our goal with building this dataset was to test out robustness and generalizability in computer vision tasks. As a result, the focus was on gathering large quantities of data from different publicly-available internet data sources. The data was gathered in a mostly non-interventionist manner. However, we only crawled websites that had policies against excessively violent and adult images and allowed us to filter out such content. We do not intend for this dataset to be used as the basis for any commercial or deployed model and will not be releasing the dataset.
## Performance and Limitations
### Performance
We have evaluated the performance of CLIP on a wide range of benchmarks across a variety of computer vision datasets such as OCR to texture recognition to fine-grained classification. The paper describes model performance on the following datasets:
- Food101
- CIFAR10
- CIFAR100
- Birdsnap
- SUN397
- Stanford Cars
- FGVC Aircraft
- VOC2007
- DTD
- Oxford-IIIT Pet dataset
- Caltech101
- Flowers102
- MNIST
- SVHN
- IIIT5K
- Hateful Memes
- SST-2
- UCF101
- Kinetics700
- Country211
- CLEVR Counting
- KITTI Distance
- STL-10
- RareAct
- Flickr30
- MSCOCO
- ImageNet
- ImageNet-A
- ImageNet-R
- ImageNet Sketch
- ObjectNet (ImageNet Overlap)
- Youtube-BB
- ImageNet-Vid
## Limitations
CLIP and our analysis of it have a number of limitations. CLIP currently struggles with respect to certain tasks such as fine grained classification and counting objects. CLIP also poses issues with regards to fairness and bias which we discuss in the paper and briefly in the next section. Additionally, our approach to testing CLIP also has an important limitation- in many cases we have used linear probes to evaluate the performance of CLIP and there is evidence suggesting that linear probes can underestimate model performance.
### Bias and Fairness
We find that the performance of CLIP - and the specific biases it exhibits - can depend significantly on class design and the choices one makes for categories to include and exclude. We tested the risk of certain kinds of denigration with CLIP by classifying images of people from [Fairface](https://arxiv.org/abs/1908.04913) into crime-related and non-human animal categories. We found significant disparities with respect to race and gender. Additionally, we found that these disparities could shift based on how the classes were constructed. (Details captured in the Broader Impacts Section in the paper).
We also tested the performance of CLIP on gender, race and age classification using the Fairface dataset (We default to using race categories as they are constructed in the Fairface dataset.) in order to assess quality of performance across different demographics. We found accuracy >96% across all races for gender classification with ‘Middle Eastern’ having the highest accuracy (98.4%) and ‘White’ having the lowest (96.5%). Additionally, CLIP averaged ~93% for racial classification and ~63% for age classification. Our use of evaluations to test for gender, race and age classification as well as denigration harms is simply to evaluate performance of the model across people and surface potential risks and not to demonstrate an endorsement/enthusiasm for such tasks.
## Feedback
### Where to send questions or comments about the model
Please use [this Google Form](https://forms.gle/Uv7afRH5dvY34ZEs9)
|
FacebookAI/roberta-base | FacebookAI | "2024-02-19T12:39:28" | 21,880,494 | 431 | transformers | [
"transformers",
"pytorch",
"tf",
"jax",
"rust",
"safetensors",
"roberta",
"fill-mask",
"exbert",
"en",
"dataset:bookcorpus",
"dataset:wikipedia",
"arxiv:1907.11692",
"arxiv:1806.02847",
"license:mit",
"autotrain_compatible",
"endpoints_compatible",
"region:us"
] | fill-mask | "2022-03-02T23:29:04" | ---
language: en
tags:
- exbert
license: mit
datasets:
- bookcorpus
- wikipedia
---
# RoBERTa base model
Pretrained model on English language using a masked language modeling (MLM) objective. It was introduced in
[this paper](https://arxiv.org/abs/1907.11692) and first released in
[this repository](https://github.com/pytorch/fairseq/tree/master/examples/roberta). This model is case-sensitive: it
makes a difference between english and English.
Disclaimer: The team releasing RoBERTa did not write a model card for this model so this model card has been written by
the Hugging Face team.
## Model description
RoBERTa is a transformers model pretrained on a large corpus of English data in a self-supervised fashion. This means
it was pretrained on the raw texts only, with no humans labelling them in any way (which is why it can use lots of
publicly available data) with an automatic process to generate inputs and labels from those texts.
More precisely, it was pretrained with the Masked language modeling (MLM) objective. Taking a sentence, the model
randomly masks 15% of the words in the input then run the entire masked sentence through the model and has to predict
the masked words. This is different from traditional recurrent neural networks (RNNs) that usually see the words one
after the other, or from autoregressive models like GPT which internally mask the future tokens. It allows the model to
learn a bidirectional representation of the sentence.
This way, the model learns an inner representation of the English language that can then be used to extract features
useful for downstream tasks: if you have a dataset of labeled sentences for instance, you can train a standard
classifier using the features produced by the BERT model as inputs.
## Intended uses & limitations
You can use the raw model for masked language modeling, but it's mostly intended to be fine-tuned on a downstream task.
See the [model hub](https://huggingface.co/models?filter=roberta) to look for fine-tuned versions on a task that
interests you.
Note that this model is primarily aimed at being fine-tuned on tasks that use the whole sentence (potentially masked)
to make decisions, such as sequence classification, token classification or question answering. For tasks such as text
generation you should look at a model like GPT2.
### How to use
You can use this model directly with a pipeline for masked language modeling:
```python
>>> from transformers import pipeline
>>> unmasker = pipeline('fill-mask', model='roberta-base')
>>> unmasker("Hello I'm a <mask> model.")
[{'sequence': "<s>Hello I'm a male model.</s>",
'score': 0.3306540250778198,
'token': 2943,
'token_str': 'Ġmale'},
{'sequence': "<s>Hello I'm a female model.</s>",
'score': 0.04655390977859497,
'token': 2182,
'token_str': 'Ġfemale'},
{'sequence': "<s>Hello I'm a professional model.</s>",
'score': 0.04232972860336304,
'token': 2038,
'token_str': 'Ġprofessional'},
{'sequence': "<s>Hello I'm a fashion model.</s>",
'score': 0.037216778844594955,
'token': 2734,
'token_str': 'Ġfashion'},
{'sequence': "<s>Hello I'm a Russian model.</s>",
'score': 0.03253649175167084,
'token': 1083,
'token_str': 'ĠRussian'}]
```
Here is how to use this model to get the features of a given text in PyTorch:
```python
from transformers import RobertaTokenizer, RobertaModel
tokenizer = RobertaTokenizer.from_pretrained('roberta-base')
model = RobertaModel.from_pretrained('roberta-base')
text = "Replace me by any text you'd like."
encoded_input = tokenizer(text, return_tensors='pt')
output = model(**encoded_input)
```
and in TensorFlow:
```python
from transformers import RobertaTokenizer, TFRobertaModel
tokenizer = RobertaTokenizer.from_pretrained('roberta-base')
model = TFRobertaModel.from_pretrained('roberta-base')
text = "Replace me by any text you'd like."
encoded_input = tokenizer(text, return_tensors='tf')
output = model(encoded_input)
```
### Limitations and bias
The training data used for this model contains a lot of unfiltered content from the internet, which is far from
neutral. Therefore, the model can have biased predictions:
```python
>>> from transformers import pipeline
>>> unmasker = pipeline('fill-mask', model='roberta-base')
>>> unmasker("The man worked as a <mask>.")
[{'sequence': '<s>The man worked as a mechanic.</s>',
'score': 0.08702439814805984,
'token': 25682,
'token_str': 'Ġmechanic'},
{'sequence': '<s>The man worked as a waiter.</s>',
'score': 0.0819653645157814,
'token': 38233,
'token_str': 'Ġwaiter'},
{'sequence': '<s>The man worked as a butcher.</s>',
'score': 0.073323555290699,
'token': 32364,
'token_str': 'Ġbutcher'},
{'sequence': '<s>The man worked as a miner.</s>',
'score': 0.046322137117385864,
'token': 18678,
'token_str': 'Ġminer'},
{'sequence': '<s>The man worked as a guard.</s>',
'score': 0.040150221437215805,
'token': 2510,
'token_str': 'Ġguard'}]
>>> unmasker("The Black woman worked as a <mask>.")
[{'sequence': '<s>The Black woman worked as a waitress.</s>',
'score': 0.22177888453006744,
'token': 35698,
'token_str': 'Ġwaitress'},
{'sequence': '<s>The Black woman worked as a prostitute.</s>',
'score': 0.19288744032382965,
'token': 36289,
'token_str': 'Ġprostitute'},
{'sequence': '<s>The Black woman worked as a maid.</s>',
'score': 0.06498628109693527,
'token': 29754,
'token_str': 'Ġmaid'},
{'sequence': '<s>The Black woman worked as a secretary.</s>',
'score': 0.05375480651855469,
'token': 2971,
'token_str': 'Ġsecretary'},
{'sequence': '<s>The Black woman worked as a nurse.</s>',
'score': 0.05245552211999893,
'token': 9008,
'token_str': 'Ġnurse'}]
```
This bias will also affect all fine-tuned versions of this model.
## Training data
The RoBERTa model was pretrained on the reunion of five datasets:
- [BookCorpus](https://yknzhu.wixsite.com/mbweb), a dataset consisting of 11,038 unpublished books;
- [English Wikipedia](https://en.wikipedia.org/wiki/English_Wikipedia) (excluding lists, tables and headers) ;
- [CC-News](https://commoncrawl.org/2016/10/news-dataset-available/), a dataset containing 63 millions English news
articles crawled between September 2016 and February 2019.
- [OpenWebText](https://github.com/jcpeterson/openwebtext), an opensource recreation of the WebText dataset used to
train GPT-2,
- [Stories](https://arxiv.org/abs/1806.02847) a dataset containing a subset of CommonCrawl data filtered to match the
story-like style of Winograd schemas.
Together these datasets weigh 160GB of text.
## Training procedure
### Preprocessing
The texts are tokenized using a byte version of Byte-Pair Encoding (BPE) and a vocabulary size of 50,000. The inputs of
the model take pieces of 512 contiguous tokens that may span over documents. The beginning of a new document is marked
with `<s>` and the end of one by `</s>`
The details of the masking procedure for each sentence are the following:
- 15% of the tokens are masked.
- In 80% of the cases, the masked tokens are replaced by `<mask>`.
- In 10% of the cases, the masked tokens are replaced by a random token (different) from the one they replace.
- In the 10% remaining cases, the masked tokens are left as is.
Contrary to BERT, the masking is done dynamically during pretraining (e.g., it changes at each epoch and is not fixed).
### Pretraining
The model was trained on 1024 V100 GPUs for 500K steps with a batch size of 8K and a sequence length of 512. The
optimizer used is Adam with a learning rate of 6e-4, \\(\beta_{1} = 0.9\\), \\(\beta_{2} = 0.98\\) and
\\(\epsilon = 1e-6\\), a weight decay of 0.01, learning rate warmup for 24,000 steps and linear decay of the learning
rate after.
## Evaluation results
When fine-tuned on downstream tasks, this model achieves the following results:
Glue test results:
| Task | MNLI | QQP | QNLI | SST-2 | CoLA | STS-B | MRPC | RTE |
|:----:|:----:|:----:|:----:|:-----:|:----:|:-----:|:----:|:----:|
| | 87.6 | 91.9 | 92.8 | 94.8 | 63.6 | 91.2 | 90.2 | 78.7 |
### BibTeX entry and citation info
```bibtex
@article{DBLP:journals/corr/abs-1907-11692,
author = {Yinhan Liu and
Myle Ott and
Naman Goyal and
Jingfei Du and
Mandar Joshi and
Danqi Chen and
Omer Levy and
Mike Lewis and
Luke Zettlemoyer and
Veselin Stoyanov},
title = {RoBERTa: {A} Robustly Optimized {BERT} Pretraining Approach},
journal = {CoRR},
volume = {abs/1907.11692},
year = {2019},
url = {http://arxiv.org/abs/1907.11692},
archivePrefix = {arXiv},
eprint = {1907.11692},
timestamp = {Thu, 01 Aug 2019 08:59:33 +0200},
biburl = {https://dblp.org/rec/journals/corr/abs-1907-11692.bib},
bibsource = {dblp computer science bibliography, https://dblp.org}
}
```
<a href="https://huggingface.co/exbert/?model=roberta-base">
<img width="300px" src="https://cdn-media.huggingface.co/exbert/button.png">
</a>
|
nesaorg/fc_6 | nesaorg | "2024-08-14T12:56:21" | 21,145,609 | 0 | null | [
"safetensors",
"model_hub_mixin",
"pytorch_model_hub_mixin",
"region:us"
] | null | "2024-08-14T12:56:03" | ---
tags:
- model_hub_mixin
- pytorch_model_hub_mixin
---
This model has been pushed to the Hub using the [PytorchModelHubMixin](https://huggingface.co/docs/huggingface_hub/package_reference/mixins#huggingface_hub.PyTorchModelHubMixin) integration:
- Library: [More Information Needed]
- Docs: [More Information Needed] |
Qwen/Qwen2.5-1.5B-Instruct | Qwen | "2024-09-25T12:32:50" | 18,826,792 | 132 | transformers | [
"transformers",
"safetensors",
"qwen2",
"text-generation",
"chat",
"conversational",
"en",
"arxiv:2407.10671",
"base_model:Qwen/Qwen2.5-1.5B",
"base_model:finetune:Qwen/Qwen2.5-1.5B",
"license:apache-2.0",
"autotrain_compatible",
"text-generation-inference",
"endpoints_compatible",
"region:us"
] | text-generation | "2024-09-17T14:10:29" | ---
license: apache-2.0
license_link: https://huggingface.co/Qwen/Qwen2.5-1.5B-Instruct/blob/main/LICENSE
language:
- en
pipeline_tag: text-generation
base_model: Qwen/Qwen2.5-1.5B
tags:
- chat
library_name: transformers
---
# Qwen2.5-1.5B-Instruct
## Introduction
Qwen2.5 is the latest series of Qwen large language models. For Qwen2.5, we release a number of base language models and instruction-tuned language models ranging from 0.5 to 72 billion parameters. Qwen2.5 brings the following improvements upon Qwen2:
- Significantly **more knowledge** and has greatly improved capabilities in **coding** and **mathematics**, thanks to our specialized expert models in these domains.
- Significant improvements in **instruction following**, **generating long texts** (over 8K tokens), **understanding structured data** (e.g, tables), and **generating structured outputs** especially JSON. **More resilient to the diversity of system prompts**, enhancing role-play implementation and condition-setting for chatbots.
- **Long-context Support** up to 128K tokens and can generate up to 8K tokens.
- **Multilingual support** for over 29 languages, including Chinese, English, French, Spanish, Portuguese, German, Italian, Russian, Japanese, Korean, Vietnamese, Thai, Arabic, and more.
**This repo contains the instruction-tuned 1.5B Qwen2.5 model**, which has the following features:
- Type: Causal Language Models
- Training Stage: Pretraining & Post-training
- Architecture: transformers with RoPE, SwiGLU, RMSNorm, Attention QKV bias and tied word embeddings
- Number of Parameters: 1.54B
- Number of Paramaters (Non-Embedding): 1.31B
- Number of Layers: 28
- Number of Attention Heads (GQA): 12 for Q and 2 for KV
- Context Length: Full 32,768 tokens and generation 8192 tokens
For more details, please refer to our [blog](https://qwenlm.github.io/blog/qwen2.5/), [GitHub](https://github.com/QwenLM/Qwen2.5), and [Documentation](https://qwen.readthedocs.io/en/latest/).
## Requirements
The code of Qwen2.5 has been in the latest Hugging face `transformers` and we advise you to use the latest version of `transformers`.
With `transformers<4.37.0`, you will encounter the following error:
```
KeyError: 'qwen2'
```
## Quickstart
Here provides a code snippet with `apply_chat_template` to show you how to load the tokenizer and model and how to generate contents.
```python
from transformers import AutoModelForCausalLM, AutoTokenizer
model_name = "Qwen/Qwen2.5-1.5B-Instruct"
model = AutoModelForCausalLM.from_pretrained(
model_name,
torch_dtype="auto",
device_map="auto"
)
tokenizer = AutoTokenizer.from_pretrained(model_name)
prompt = "Give me a short introduction to large language model."
messages = [
{"role": "system", "content": "You are Qwen, created by Alibaba Cloud. You are a helpful assistant."},
{"role": "user", "content": prompt}
]
text = tokenizer.apply_chat_template(
messages,
tokenize=False,
add_generation_prompt=True
)
model_inputs = tokenizer([text], return_tensors="pt").to(model.device)
generated_ids = model.generate(
**model_inputs,
max_new_tokens=512
)
generated_ids = [
output_ids[len(input_ids):] for input_ids, output_ids in zip(model_inputs.input_ids, generated_ids)
]
response = tokenizer.batch_decode(generated_ids, skip_special_tokens=True)[0]
```
## Evaluation & Performance
Detailed evaluation results are reported in this [📑 blog](https://qwenlm.github.io/blog/qwen2.5/).
For requirements on GPU memory and the respective throughput, see results [here](https://qwen.readthedocs.io/en/latest/benchmark/speed_benchmark.html).
## Citation
If you find our work helpful, feel free to give us a cite.
```
@misc{qwen2.5,
title = {Qwen2.5: A Party of Foundation Models},
url = {https://qwenlm.github.io/blog/qwen2.5/},
author = {Qwen Team},
month = {September},
year = {2024}
}
@article{qwen2,
title={Qwen2 Technical Report},
author={An Yang and Baosong Yang and Binyuan Hui and Bo Zheng and Bowen Yu and Chang Zhou and Chengpeng Li and Chengyuan Li and Dayiheng Liu and Fei Huang and Guanting Dong and Haoran Wei and Huan Lin and Jialong Tang and Jialin Wang and Jian Yang and Jianhong Tu and Jianwei Zhang and Jianxin Ma and Jin Xu and Jingren Zhou and Jinze Bai and Jinzheng He and Junyang Lin and Kai Dang and Keming Lu and Keqin Chen and Kexin Yang and Mei Li and Mingfeng Xue and Na Ni and Pei Zhang and Peng Wang and Ru Peng and Rui Men and Ruize Gao and Runji Lin and Shijie Wang and Shuai Bai and Sinan Tan and Tianhang Zhu and Tianhao Li and Tianyu Liu and Wenbin Ge and Xiaodong Deng and Xiaohuan Zhou and Xingzhang Ren and Xinyu Zhang and Xipin Wei and Xuancheng Ren and Yang Fan and Yang Yao and Yichang Zhang and Yu Wan and Yunfei Chu and Yuqiong Liu and Zeyu Cui and Zhenru Zhang and Zhihao Fan},
journal={arXiv preprint arXiv:2407.10671},
year={2024}
}
``` |
facebook/opt-1.3b | facebook | "2023-09-15T13:09:33" | 18,756,029 | 154 | transformers | [
"transformers",
"pytorch",
"tf",
"jax",
"opt",
"text-generation",
"en",
"arxiv:2205.01068",
"arxiv:2005.14165",
"license:other",
"autotrain_compatible",
"text-generation-inference",
"region:us"
] | text-generation | "2022-05-11T08:26:00" | ---
language: en
inference: false
tags:
- text-generation
- opt
license: other
commercial: false
---
# OPT : Open Pre-trained Transformer Language Models
OPT was first introduced in [Open Pre-trained Transformer Language Models](https://arxiv.org/abs/2205.01068) and first released in [metaseq's repository](https://github.com/facebookresearch/metaseq) on May 3rd 2022 by Meta AI.
**Disclaimer**: The team releasing OPT wrote an official model card, which is available in Appendix D of the [paper](https://arxiv.org/pdf/2205.01068.pdf).
Content from **this** model card has been written by the Hugging Face team.
## Intro
To quote the first two paragraphs of the [official paper](https://arxiv.org/abs/2205.01068)
> Large language models trained on massive text collections have shown surprising emergent
> capabilities to generate text and perform zero- and few-shot learning. While in some cases the public
> can interact with these models through paid APIs, full model access is currently limited to only a
> few highly resourced labs. This restricted access has limited researchers’ ability to study how and
> why these large language models work, hindering progress on improving known challenges in areas
> such as robustness, bias, and toxicity.
> We present Open Pretrained Transformers (OPT), a suite of decoder-only pre-trained transformers ranging from 125M
> to 175B parameters, which we aim to fully and responsibly share with interested researchers. We train the OPT models to roughly match
> the performance and sizes of the GPT-3 class of models, while also applying the latest best practices in data
> collection and efficient training. Our aim in developing this suite of OPT models is to enable reproducible and responsible research at scale, and
> to bring more voices to the table in studying the impact of these LLMs. Definitions of risk, harm, bias, and toxicity, etc., should be articulated by the
> collective research community as a whole, which is only possible when models are available for study.
## Model description
OPT was predominantly pretrained with English text, but a small amount of non-English data is still present within the training corpus via CommonCrawl. The model was pretrained using a causal language modeling (CLM) objective.
OPT belongs to the same family of decoder-only models like [GPT-3](https://arxiv.org/abs/2005.14165). As such, it was pretrained using the self-supervised causal language modedling objective.
For evaluation, OPT follows [GPT-3](https://arxiv.org/abs/2005.14165) by using their prompts and overall experimental setup. For more details, please read
the [official paper](https://arxiv.org/abs/2205.01068).
## Intended uses & limitations
The pretrained-only model can be used for prompting for evaluation of downstream tasks as well as text generation.
In addition, the model can be fine-tuned on a downstream task using the [CLM example](https://github.com/huggingface/transformers/tree/main/examples/pytorch/language-modeling). For all other OPT checkpoints, please have a look at the [model hub](https://huggingface.co/models?filter=opt).
### How to use
You can use this model directly with a pipeline for text generation.
```python
>>> from transformers import pipeline
>>> generator = pipeline('text-generation', model="facebook/opt-1.3b")
>>> generator("What are we having for dinner?")
[{'generated_text': 'What are we having for dinner?\nI'm not sure. I'm not a chef. I'}]
```
By default, generation is deterministic. In order to use the top-k sampling, please set `do_sample` to `True`.
```python
>>> from transformers import pipeline, set_seed
>>> set_seed(32)
>>> generator = pipeline('text-generation', model="facebook/opt-1.3b", do_sample=True)
>>> generator("What are we having for dinner?")
[{'generated_text': "What are we having for dinner?\nI have had chicken and rice for lunch. It is delicious"}]
```
### Limitations and bias
As mentioned in Meta AI's model card, given that the training data used for this model contains a lot of
unfiltered content from the internet, which is far from neutral the model is strongly biased :
> Like other large language models for which the diversity (or lack thereof) of training
> data induces downstream impact on the quality of our model, OPT-175B has limitations in terms
> of bias and safety. OPT-175B can also have quality issues in terms of generation diversity and
> hallucination. In general, OPT-175B is not immune from the plethora of issues that plague modern
> large language models.
Here's an example of how the model can have biased predictions:
```python
>>> from transformers import pipeline, set_seed
>>> set_seed(32)
>>> generator = pipeline('text-generation', model="facebook/opt-1.3b", do_sample=True, num_return_sequences=5)
>>> generator("The woman worked as a")
[{'generated_text': 'The woman worked as a bartender for six months before getting to the job she always dreamed of. She'},
{'generated_text': 'The woman worked as a nanny in a house near The White Horse Farm in the Yorkshire Dales'},
{'generated_text': "The woman worked as a translator at the British Broadcasting Corporation's headquarters and was also an acquaintance of some"},
{'generated_text': 'The woman worked as a secretary and went to school full-time, and also worked as a waitress'},
{'generated_text': 'The woman worked as a beautician with her baby and the little girl is now at the age where'}]
```
compared to:
```python
>>> from transformers import pipeline, set_seed
>>> set_seed(32)
>>> generator = pipeline('text-generation', model="facebook/opt-1.3b", do_sample=True, num_return_sequences=5)
>>> generator("The man worked as a")
[{'generated_text': 'The man worked as a janitor and the owner of the house he worked at caught him cheating on'},
{'generated_text': 'The man worked as a software engineer.\n\nFor over 10 years, he had been at Amazon'},
{'generated_text': 'The man worked as a car salesman - and was a man of his word to her\nA T'},
{'generated_text': 'The man worked as a private contractor for five years. He went to the Bahamas in the summer of'},
{'generated_text': 'The man worked as a computer systems consultant. After leaving the job, he became a prolific internet hacker'}]
```
This bias will also affect all fine-tuned versions of this model.
## Training data
The Meta AI team wanted to train this model on a corpus as large as possible. It is composed of the union of the following 5 filtered datasets of textual documents:
- BookCorpus, which consists of more than 10K unpublished books,
- CC-Stories, which contains a subset of CommonCrawl data filtered to match the
story-like style of Winograd schemas,
- The Pile, from which * Pile-CC, OpenWebText2, USPTO, Project Gutenberg, OpenSubtitles, Wikipedia, DM Mathematics and HackerNews* were included.
- Pushshift.io Reddit dataset that was developed in Baumgartner et al. (2020) and processed in
Roller et al. (2021)
- CCNewsV2 containing an updated version of the English portion of the CommonCrawl News
dataset that was used in RoBERTa (Liu et al., 2019b)
The final training data contains 180B tokens corresponding to 800GB of data. The validation split was made of 200MB of the pretraining data, sampled proportionally
to each dataset’s size in the pretraining corpus.
The dataset might contains offensive content as parts of the dataset are a subset of
public Common Crawl data, along with a subset of public Reddit data, which could contain sentences
that, if viewed directly, can be insulting, threatening, or might otherwise cause anxiety.
### Collection process
The dataset was collected form internet, and went through classic data processing algorithms and
re-formatting practices, including removing repetitive/non-informative text like *Chapter One* or
*This ebook by Project Gutenberg.*
## Training procedure
### Preprocessing
The texts are tokenized using the **GPT2** byte-level version of Byte Pair Encoding (BPE) (for unicode characters) and a
vocabulary size of 50272. The inputs are sequences of 2048 consecutive tokens.
The 175B model was trained on 992 *80GB A100 GPUs*. The training duration was roughly ~33 days of continuous training.
### BibTeX entry and citation info
```bibtex
@misc{zhang2022opt,
title={OPT: Open Pre-trained Transformer Language Models},
author={Susan Zhang and Stephen Roller and Naman Goyal and Mikel Artetxe and Moya Chen and Shuohui Chen and Christopher Dewan and Mona Diab and Xian Li and Xi Victoria Lin and Todor Mihaylov and Myle Ott and Sam Shleifer and Kurt Shuster and Daniel Simig and Punit Singh Koura and Anjali Sridhar and Tianlu Wang and Luke Zettlemoyer},
year={2022},
eprint={2205.01068},
archivePrefix={arXiv},
primaryClass={cs.CL}
}
``` |
google/vit-base-patch16-224-in21k | google | "2024-02-05T16:37:39" | 17,584,655 | 245 | transformers | [
"transformers",
"pytorch",
"tf",
"jax",
"safetensors",
"vit",
"image-feature-extraction",
"vision",
"dataset:imagenet-21k",
"arxiv:2010.11929",
"arxiv:2006.03677",
"license:apache-2.0",
"region:us"
] | image-feature-extraction | "2022-03-02T23:29:05" | ---
license: apache-2.0
tags:
- vision
datasets:
- imagenet-21k
inference: false
---
# Vision Transformer (base-sized model)
Vision Transformer (ViT) model pre-trained on ImageNet-21k (14 million images, 21,843 classes) at resolution 224x224. It was introduced in the paper [An Image is Worth 16x16 Words: Transformers for Image Recognition at Scale](https://arxiv.org/abs/2010.11929) by Dosovitskiy et al. and first released in [this repository](https://github.com/google-research/vision_transformer). However, the weights were converted from the [timm repository](https://github.com/rwightman/pytorch-image-models) by Ross Wightman, who already converted the weights from JAX to PyTorch. Credits go to him.
Disclaimer: The team releasing ViT did not write a model card for this model so this model card has been written by the Hugging Face team.
## Model description
The Vision Transformer (ViT) is a transformer encoder model (BERT-like) pretrained on a large collection of images in a supervised fashion, namely ImageNet-21k, at a resolution of 224x224 pixels.
Images are presented to the model as a sequence of fixed-size patches (resolution 16x16), which are linearly embedded. One also adds a [CLS] token to the beginning of a sequence to use it for classification tasks. One also adds absolute position embeddings before feeding the sequence to the layers of the Transformer encoder.
Note that this model does not provide any fine-tuned heads, as these were zero'd by Google researchers. However, the model does include the pre-trained pooler, which can be used for downstream tasks (such as image classification).
By pre-training the model, it learns an inner representation of images that can then be used to extract features useful for downstream tasks: if you have a dataset of labeled images for instance, you can train a standard classifier by placing a linear layer on top of the pre-trained encoder. One typically places a linear layer on top of the [CLS] token, as the last hidden state of this token can be seen as a representation of an entire image.
## Intended uses & limitations
You can use the raw model for image classification. See the [model hub](https://huggingface.co/models?search=google/vit) to look for
fine-tuned versions on a task that interests you.
### How to use
Here is how to use this model in PyTorch:
```python
from transformers import ViTImageProcessor, ViTModel
from PIL import Image
import requests
url = 'http://images.cocodataset.org/val2017/000000039769.jpg'
image = Image.open(requests.get(url, stream=True).raw)
processor = ViTImageProcessor.from_pretrained('google/vit-base-patch16-224-in21k')
model = ViTModel.from_pretrained('google/vit-base-patch16-224-in21k')
inputs = processor(images=image, return_tensors="pt")
outputs = model(**inputs)
last_hidden_states = outputs.last_hidden_state
```
Here is how to use this model in JAX/Flax:
```python
from transformers import ViTImageProcessor, FlaxViTModel
from PIL import Image
import requests
url = 'http://images.cocodataset.org/val2017/000000039769.jpg'
image = Image.open(requests.get(url, stream=True).raw)
processor = ViTImageProcessor.from_pretrained('google/vit-base-patch16-224-in21k')
model = FlaxViTModel.from_pretrained('google/vit-base-patch16-224-in21k')
inputs = processor(images=image, return_tensors="np")
outputs = model(**inputs)
last_hidden_states = outputs.last_hidden_state
```
## Training data
The ViT model was pretrained on [ImageNet-21k](http://www.image-net.org/), a dataset consisting of 14 million images and 21k classes.
## Training procedure
### Preprocessing
The exact details of preprocessing of images during training/validation can be found [here](https://github.com/google-research/vision_transformer/blob/master/vit_jax/input_pipeline.py).
Images are resized/rescaled to the same resolution (224x224) and normalized across the RGB channels with mean (0.5, 0.5, 0.5) and standard deviation (0.5, 0.5, 0.5).
### Pretraining
The model was trained on TPUv3 hardware (8 cores). All model variants are trained with a batch size of 4096 and learning rate warmup of 10k steps. For ImageNet, the authors found it beneficial to additionally apply gradient clipping at global norm 1. Pre-training resolution is 224.
## Evaluation results
For evaluation results on several image classification benchmarks, we refer to tables 2 and 5 of the original paper. Note that for fine-tuning, the best results are obtained with a higher resolution (384x384). Of course, increasing the model size will result in better performance.
### BibTeX entry and citation info
```bibtex
@misc{wu2020visual,
title={Visual Transformers: Token-based Image Representation and Processing for Computer Vision},
author={Bichen Wu and Chenfeng Xu and Xiaoliang Dai and Alvin Wan and Peizhao Zhang and Zhicheng Yan and Masayoshi Tomizuka and Joseph Gonzalez and Kurt Keutzer and Peter Vajda},
year={2020},
eprint={2006.03677},
archivePrefix={arXiv},
primaryClass={cs.CV}
}
```
```bibtex
@inproceedings{deng2009imagenet,
title={Imagenet: A large-scale hierarchical image database},
author={Deng, Jia and Dong, Wei and Socher, Richard and Li, Li-Jia and Li, Kai and Fei-Fei, Li},
booktitle={2009 IEEE conference on computer vision and pattern recognition},
pages={248--255},
year={2009},
organization={Ieee}
}
``` |
openai-community/gpt2 | openai-community | "2024-02-19T10:57:45" | 17,116,852 | 2,356 | transformers | [
"transformers",
"pytorch",
"tf",
"jax",
"tflite",
"rust",
"onnx",
"safetensors",
"gpt2",
"text-generation",
"exbert",
"en",
"doi:10.57967/hf/0039",
"license:mit",
"autotrain_compatible",
"text-generation-inference",
"endpoints_compatible",
"region:us"
] | text-generation | "2022-03-02T23:29:04" | ---
language: en
tags:
- exbert
license: mit
---
# GPT-2
Test the whole generation capabilities here: https://transformer.huggingface.co/doc/gpt2-large
Pretrained model on English language using a causal language modeling (CLM) objective. It was introduced in
[this paper](https://d4mucfpksywv.cloudfront.net/better-language-models/language_models_are_unsupervised_multitask_learners.pdf)
and first released at [this page](https://openai.com/blog/better-language-models/).
Disclaimer: The team releasing GPT-2 also wrote a
[model card](https://github.com/openai/gpt-2/blob/master/model_card.md) for their model. Content from this model card
has been written by the Hugging Face team to complete the information they provided and give specific examples of bias.
## Model description
GPT-2 is a transformers model pretrained on a very large corpus of English data in a self-supervised fashion. This
means it was pretrained on the raw texts only, with no humans labelling them in any way (which is why it can use lots
of publicly available data) with an automatic process to generate inputs and labels from those texts. More precisely,
it was trained to guess the next word in sentences.
More precisely, inputs are sequences of continuous text of a certain length and the targets are the same sequence,
shifted one token (word or piece of word) to the right. The model uses internally a mask-mechanism to make sure the
predictions for the token `i` only uses the inputs from `1` to `i` but not the future tokens.
This way, the model learns an inner representation of the English language that can then be used to extract features
useful for downstream tasks. The model is best at what it was pretrained for however, which is generating texts from a
prompt.
This is the **smallest** version of GPT-2, with 124M parameters.
**Related Models:** [GPT-Large](https://huggingface.co/gpt2-large), [GPT-Medium](https://huggingface.co/gpt2-medium) and [GPT-XL](https://huggingface.co/gpt2-xl)
## Intended uses & limitations
You can use the raw model for text generation or fine-tune it to a downstream task. See the
[model hub](https://huggingface.co/models?filter=gpt2) to look for fine-tuned versions on a task that interests you.
### How to use
You can use this model directly with a pipeline for text generation. Since the generation relies on some randomness, we
set a seed for reproducibility:
```python
>>> from transformers import pipeline, set_seed
>>> generator = pipeline('text-generation', model='gpt2')
>>> set_seed(42)
>>> generator("Hello, I'm a language model,", max_length=30, num_return_sequences=5)
[{'generated_text': "Hello, I'm a language model, a language for thinking, a language for expressing thoughts."},
{'generated_text': "Hello, I'm a language model, a compiler, a compiler library, I just want to know how I build this kind of stuff. I don"},
{'generated_text': "Hello, I'm a language model, and also have more than a few of your own, but I understand that they're going to need some help"},
{'generated_text': "Hello, I'm a language model, a system model. I want to know my language so that it might be more interesting, more user-friendly"},
{'generated_text': 'Hello, I\'m a language model, not a language model"\n\nThe concept of "no-tricks" comes in handy later with new'}]
```
Here is how to use this model to get the features of a given text in PyTorch:
```python
from transformers import GPT2Tokenizer, GPT2Model
tokenizer = GPT2Tokenizer.from_pretrained('gpt2')
model = GPT2Model.from_pretrained('gpt2')
text = "Replace me by any text you'd like."
encoded_input = tokenizer(text, return_tensors='pt')
output = model(**encoded_input)
```
and in TensorFlow:
```python
from transformers import GPT2Tokenizer, TFGPT2Model
tokenizer = GPT2Tokenizer.from_pretrained('gpt2')
model = TFGPT2Model.from_pretrained('gpt2')
text = "Replace me by any text you'd like."
encoded_input = tokenizer(text, return_tensors='tf')
output = model(encoded_input)
```
### Limitations and bias
The training data used for this model has not been released as a dataset one can browse. We know it contains a lot of
unfiltered content from the internet, which is far from neutral. As the openAI team themselves point out in their
[model card](https://github.com/openai/gpt-2/blob/master/model_card.md#out-of-scope-use-cases):
> Because large-scale language models like GPT-2 do not distinguish fact from fiction, we don’t support use-cases
> that require the generated text to be true.
>
> Additionally, language models like GPT-2 reflect the biases inherent to the systems they were trained on, so we do
> not recommend that they be deployed into systems that interact with humans > unless the deployers first carry out a
> study of biases relevant to the intended use-case. We found no statistically significant difference in gender, race,
> and religious bias probes between 774M and 1.5B, implying all versions of GPT-2 should be approached with similar
> levels of caution around use cases that are sensitive to biases around human attributes.
Here's an example of how the model can have biased predictions:
```python
>>> from transformers import pipeline, set_seed
>>> generator = pipeline('text-generation', model='gpt2')
>>> set_seed(42)
>>> generator("The White man worked as a", max_length=10, num_return_sequences=5)
[{'generated_text': 'The White man worked as a mannequin for'},
{'generated_text': 'The White man worked as a maniser of the'},
{'generated_text': 'The White man worked as a bus conductor by day'},
{'generated_text': 'The White man worked as a plumber at the'},
{'generated_text': 'The White man worked as a journalist. He had'}]
>>> set_seed(42)
>>> generator("The Black man worked as a", max_length=10, num_return_sequences=5)
[{'generated_text': 'The Black man worked as a man at a restaurant'},
{'generated_text': 'The Black man worked as a car salesman in a'},
{'generated_text': 'The Black man worked as a police sergeant at the'},
{'generated_text': 'The Black man worked as a man-eating monster'},
{'generated_text': 'The Black man worked as a slave, and was'}]
```
This bias will also affect all fine-tuned versions of this model.
## Training data
The OpenAI team wanted to train this model on a corpus as large as possible. To build it, they scraped all the web
pages from outbound links on Reddit which received at least 3 karma. Note that all Wikipedia pages were removed from
this dataset, so the model was not trained on any part of Wikipedia. The resulting dataset (called WebText) weights
40GB of texts but has not been publicly released. You can find a list of the top 1,000 domains present in WebText
[here](https://github.com/openai/gpt-2/blob/master/domains.txt).
## Training procedure
### Preprocessing
The texts are tokenized using a byte-level version of Byte Pair Encoding (BPE) (for unicode characters) and a
vocabulary size of 50,257. The inputs are sequences of 1024 consecutive tokens.
The larger model was trained on 256 cloud TPU v3 cores. The training duration was not disclosed, nor were the exact
details of training.
## Evaluation results
The model achieves the following results without any fine-tuning (zero-shot):
| Dataset | LAMBADA | LAMBADA | CBT-CN | CBT-NE | WikiText2 | PTB | enwiki8 | text8 | WikiText103 | 1BW |
|:--------:|:-------:|:-------:|:------:|:------:|:---------:|:------:|:-------:|:------:|:-----------:|:-----:|
| (metric) | (PPL) | (ACC) | (ACC) | (ACC) | (PPL) | (PPL) | (BPB) | (BPC) | (PPL) | (PPL) |
| | 35.13 | 45.99 | 87.65 | 83.4 | 29.41 | 65.85 | 1.16 | 1,17 | 37.50 | 75.20 |
### BibTeX entry and citation info
```bibtex
@article{radford2019language,
title={Language Models are Unsupervised Multitask Learners},
author={Radford, Alec and Wu, Jeff and Child, Rewon and Luan, David and Amodei, Dario and Sutskever, Ilya},
year={2019}
}
```
<a href="https://huggingface.co/exbert/?model=gpt2">
<img width="300px" src="https://cdn-media.huggingface.co/exbert/button.png">
</a>
|
bigscience/bloomz-560m | bigscience | "2023-05-27T17:27:11" | 16,662,195 | 107 | transformers | [
"transformers",
"pytorch",
"tensorboard",
"safetensors",
"bloom",
"text-generation",
"ak",
"ar",
"as",
"bm",
"bn",
"ca",
"code",
"en",
"es",
"eu",
"fon",
"fr",
"gu",
"hi",
"id",
"ig",
"ki",
"kn",
"lg",
"ln",
"ml",
"mr",
"ne",
"nso",
"ny",
"or",
"pa",
"pt",
"rn",
"rw",
"sn",
"st",
"sw",
"ta",
"te",
"tn",
"ts",
"tum",
"tw",
"ur",
"vi",
"wo",
"xh",
"yo",
"zh",
"zu",
"dataset:bigscience/xP3",
"arxiv:2211.01786",
"license:bigscience-bloom-rail-1.0",
"model-index",
"autotrain_compatible",
"text-generation-inference",
"endpoints_compatible",
"region:us"
] | text-generation | "2022-10-08T16:14:42" | ---
datasets:
- bigscience/xP3
license: bigscience-bloom-rail-1.0
language:
- ak
- ar
- as
- bm
- bn
- ca
- code
- en
- es
- eu
- fon
- fr
- gu
- hi
- id
- ig
- ki
- kn
- lg
- ln
- ml
- mr
- ne
- nso
- ny
- or
- pa
- pt
- rn
- rw
- sn
- st
- sw
- ta
- te
- tn
- ts
- tum
- tw
- ur
- vi
- wo
- xh
- yo
- zh
- zu
programming_language:
- C
- C++
- C#
- Go
- Java
- JavaScript
- Lua
- PHP
- Python
- Ruby
- Rust
- Scala
- TypeScript
pipeline_tag: text-generation
widget:
- text: "一个传奇的开端,一个不灭的神话,这不仅仅是一部电影,而是作为一个走进新时代的标签,永远彪炳史册。Would you rate the previous review as positive, neutral or negative?"
example_title: "zh-en sentiment"
- text: "一个传奇的开端,一个不灭的神话,这不仅仅是一部电影,而是作为一个走进新时代的标签,永远彪炳史册。你认为这句话的立场是赞扬、中立还是批评?"
example_title: "zh-zh sentiment"
- text: "Suggest at least five related search terms to \"Mạng neural nhân tạo\"."
example_title: "vi-en query"
- text: "Proposez au moins cinq mots clés concernant «Réseau de neurones artificiels»."
example_title: "fr-fr query"
- text: "Explain in a sentence in Telugu what is backpropagation in neural networks."
example_title: "te-en qa"
- text: "Why is the sky blue?"
example_title: "en-en qa"
- text: "Write a fairy tale about a troll saving a princess from a dangerous dragon. The fairy tale is a masterpiece that has achieved praise worldwide and its moral is \"Heroes Come in All Shapes and Sizes\". Story (in Spanish):"
example_title: "es-en fable"
- text: "Write a fable about wood elves living in a forest that is suddenly invaded by ogres. The fable is a masterpiece that has achieved praise worldwide and its moral is \"Violence is the last refuge of the incompetent\". Fable (in Hindi):"
example_title: "hi-en fable"
model-index:
- name: bloomz-560m
results:
- task:
type: Coreference resolution
dataset:
type: winogrande
name: Winogrande XL (xl)
config: xl
split: validation
revision: a80f460359d1e9a67c006011c94de42a8759430c
metrics:
- type: Accuracy
value: 52.41
- task:
type: Coreference resolution
dataset:
type: Muennighoff/xwinograd
name: XWinograd (en)
config: en
split: test
revision: 9dd5ea5505fad86b7bedad667955577815300cee
metrics:
- type: Accuracy
value: 51.01
- task:
type: Coreference resolution
dataset:
type: Muennighoff/xwinograd
name: XWinograd (fr)
config: fr
split: test
revision: 9dd5ea5505fad86b7bedad667955577815300cee
metrics:
- type: Accuracy
value: 51.81
- task:
type: Coreference resolution
dataset:
type: Muennighoff/xwinograd
name: XWinograd (jp)
config: jp
split: test
revision: 9dd5ea5505fad86b7bedad667955577815300cee
metrics:
- type: Accuracy
value: 52.03
- task:
type: Coreference resolution
dataset:
type: Muennighoff/xwinograd
name: XWinograd (pt)
config: pt
split: test
revision: 9dd5ea5505fad86b7bedad667955577815300cee
metrics:
- type: Accuracy
value: 53.99
- task:
type: Coreference resolution
dataset:
type: Muennighoff/xwinograd
name: XWinograd (ru)
config: ru
split: test
revision: 9dd5ea5505fad86b7bedad667955577815300cee
metrics:
- type: Accuracy
value: 53.97
- task:
type: Coreference resolution
dataset:
type: Muennighoff/xwinograd
name: XWinograd (zh)
config: zh
split: test
revision: 9dd5ea5505fad86b7bedad667955577815300cee
metrics:
- type: Accuracy
value: 54.76
- task:
type: Natural language inference
dataset:
type: anli
name: ANLI (r1)
config: r1
split: validation
revision: 9dbd830a06fea8b1c49d6e5ef2004a08d9f45094
metrics:
- type: Accuracy
value: 33.4
- task:
type: Natural language inference
dataset:
type: anli
name: ANLI (r2)
config: r2
split: validation
revision: 9dbd830a06fea8b1c49d6e5ef2004a08d9f45094
metrics:
- type: Accuracy
value: 33.4
- task:
type: Natural language inference
dataset:
type: anli
name: ANLI (r3)
config: r3
split: validation
revision: 9dbd830a06fea8b1c49d6e5ef2004a08d9f45094
metrics:
- type: Accuracy
value: 33.5
- task:
type: Natural language inference
dataset:
type: super_glue
name: SuperGLUE (cb)
config: cb
split: validation
revision: 9e12063561e7e6c79099feb6d5a493142584e9e2
metrics:
- type: Accuracy
value: 53.57
- task:
type: Natural language inference
dataset:
type: super_glue
name: SuperGLUE (rte)
config: rte
split: validation
revision: 9e12063561e7e6c79099feb6d5a493142584e9e2
metrics:
- type: Accuracy
value: 67.15
- task:
type: Natural language inference
dataset:
type: xnli
name: XNLI (ar)
config: ar
split: validation
revision: a5a45e4ff92d5d3f34de70aaf4b72c3bdf9f7f16
metrics:
- type: Accuracy
value: 44.46
- task:
type: Natural language inference
dataset:
type: xnli
name: XNLI (bg)
config: bg
split: validation
revision: a5a45e4ff92d5d3f34de70aaf4b72c3bdf9f7f16
metrics:
- type: Accuracy
value: 39.76
- task:
type: Natural language inference
dataset:
type: xnli
name: XNLI (de)
config: de
split: validation
revision: a5a45e4ff92d5d3f34de70aaf4b72c3bdf9f7f16
metrics:
- type: Accuracy
value: 39.36
- task:
type: Natural language inference
dataset:
type: xnli
name: XNLI (el)
config: el
split: validation
revision: a5a45e4ff92d5d3f34de70aaf4b72c3bdf9f7f16
metrics:
- type: Accuracy
value: 40.96
- task:
type: Natural language inference
dataset:
type: xnli
name: XNLI (en)
config: en
split: validation
revision: a5a45e4ff92d5d3f34de70aaf4b72c3bdf9f7f16
metrics:
- type: Accuracy
value: 46.43
- task:
type: Natural language inference
dataset:
type: xnli
name: XNLI (es)
config: es
split: validation
revision: a5a45e4ff92d5d3f34de70aaf4b72c3bdf9f7f16
metrics:
- type: Accuracy
value: 44.98
- task:
type: Natural language inference
dataset:
type: xnli
name: XNLI (fr)
config: fr
split: validation
revision: a5a45e4ff92d5d3f34de70aaf4b72c3bdf9f7f16
metrics:
- type: Accuracy
value: 45.54
- task:
type: Natural language inference
dataset:
type: xnli
name: XNLI (hi)
config: hi
split: validation
revision: a5a45e4ff92d5d3f34de70aaf4b72c3bdf9f7f16
metrics:
- type: Accuracy
value: 41.81
- task:
type: Natural language inference
dataset:
type: xnli
name: XNLI (ru)
config: ru
split: validation
revision: a5a45e4ff92d5d3f34de70aaf4b72c3bdf9f7f16
metrics:
- type: Accuracy
value: 39.64
- task:
type: Natural language inference
dataset:
type: xnli
name: XNLI (sw)
config: sw
split: validation
revision: a5a45e4ff92d5d3f34de70aaf4b72c3bdf9f7f16
metrics:
- type: Accuracy
value: 38.35
- task:
type: Natural language inference
dataset:
type: xnli
name: XNLI (th)
config: th
split: validation
revision: a5a45e4ff92d5d3f34de70aaf4b72c3bdf9f7f16
metrics:
- type: Accuracy
value: 35.5
- task:
type: Natural language inference
dataset:
type: xnli
name: XNLI (tr)
config: tr
split: validation
revision: a5a45e4ff92d5d3f34de70aaf4b72c3bdf9f7f16
metrics:
- type: Accuracy
value: 37.31
- task:
type: Natural language inference
dataset:
type: xnli
name: XNLI (ur)
config: ur
split: validation
revision: a5a45e4ff92d5d3f34de70aaf4b72c3bdf9f7f16
metrics:
- type: Accuracy
value: 38.96
- task:
type: Natural language inference
dataset:
type: xnli
name: XNLI (vi)
config: vi
split: validation
revision: a5a45e4ff92d5d3f34de70aaf4b72c3bdf9f7f16
metrics:
- type: Accuracy
value: 44.74
- task:
type: Natural language inference
dataset:
type: xnli
name: XNLI (zh)
config: zh
split: validation
revision: a5a45e4ff92d5d3f34de70aaf4b72c3bdf9f7f16
metrics:
- type: Accuracy
value: 44.66
- task:
type: Program synthesis
dataset:
type: openai_humaneval
name: HumanEval
config: None
split: test
revision: e8dc562f5de170c54b5481011dd9f4fa04845771
metrics:
- type: Pass@1
value: 2.18
- type: Pass@10
value: 4.11
- type: Pass@100
value: 9.00
- task:
type: Sentence completion
dataset:
type: story_cloze
name: StoryCloze (2016)
config: "2016"
split: validation
revision: e724c6f8cdf7c7a2fb229d862226e15b023ee4db
metrics:
- type: Accuracy
value: 60.29
- task:
type: Sentence completion
dataset:
type: super_glue
name: SuperGLUE (copa)
config: copa
split: validation
revision: 9e12063561e7e6c79099feb6d5a493142584e9e2
metrics:
- type: Accuracy
value: 52.0
- task:
type: Sentence completion
dataset:
type: xcopa
name: XCOPA (et)
config: et
split: validation
revision: 37f73c60fb123111fa5af5f9b705d0b3747fd187
metrics:
- type: Accuracy
value: 53.0
- task:
type: Sentence completion
dataset:
type: xcopa
name: XCOPA (ht)
config: ht
split: validation
revision: 37f73c60fb123111fa5af5f9b705d0b3747fd187
metrics:
- type: Accuracy
value: 49.0
- task:
type: Sentence completion
dataset:
type: xcopa
name: XCOPA (id)
config: id
split: validation
revision: 37f73c60fb123111fa5af5f9b705d0b3747fd187
metrics:
- type: Accuracy
value: 57.0
- task:
type: Sentence completion
dataset:
type: xcopa
name: XCOPA (it)
config: it
split: validation
revision: 37f73c60fb123111fa5af5f9b705d0b3747fd187
metrics:
- type: Accuracy
value: 52.0
- task:
type: Sentence completion
dataset:
type: xcopa
name: XCOPA (qu)
config: qu
split: validation
revision: 37f73c60fb123111fa5af5f9b705d0b3747fd187
metrics:
- type: Accuracy
value: 55.0
- task:
type: Sentence completion
dataset:
type: xcopa
name: XCOPA (sw)
config: sw
split: validation
revision: 37f73c60fb123111fa5af5f9b705d0b3747fd187
metrics:
- type: Accuracy
value: 56.0
- task:
type: Sentence completion
dataset:
type: xcopa
name: XCOPA (ta)
config: ta
split: validation
revision: 37f73c60fb123111fa5af5f9b705d0b3747fd187
metrics:
- type: Accuracy
value: 58.0
- task:
type: Sentence completion
dataset:
type: xcopa
name: XCOPA (th)
config: th
split: validation
revision: 37f73c60fb123111fa5af5f9b705d0b3747fd187
metrics:
- type: Accuracy
value: 58.0
- task:
type: Sentence completion
dataset:
type: xcopa
name: XCOPA (tr)
config: tr
split: validation
revision: 37f73c60fb123111fa5af5f9b705d0b3747fd187
metrics:
- type: Accuracy
value: 61.0
- task:
type: Sentence completion
dataset:
type: xcopa
name: XCOPA (vi)
config: vi
split: validation
revision: 37f73c60fb123111fa5af5f9b705d0b3747fd187
metrics:
- type: Accuracy
value: 61.0
- task:
type: Sentence completion
dataset:
type: xcopa
name: XCOPA (zh)
config: zh
split: validation
revision: 37f73c60fb123111fa5af5f9b705d0b3747fd187
metrics:
- type: Accuracy
value: 61.0
- task:
type: Sentence completion
dataset:
type: Muennighoff/xstory_cloze
name: XStoryCloze (ar)
config: ar
split: validation
revision: 8bb76e594b68147f1a430e86829d07189622b90d
metrics:
- type: Accuracy
value: 54.4
- task:
type: Sentence completion
dataset:
type: Muennighoff/xstory_cloze
name: XStoryCloze (es)
config: es
split: validation
revision: 8bb76e594b68147f1a430e86829d07189622b90d
metrics:
- type: Accuracy
value: 56.45
- task:
type: Sentence completion
dataset:
type: Muennighoff/xstory_cloze
name: XStoryCloze (eu)
config: eu
split: validation
revision: 8bb76e594b68147f1a430e86829d07189622b90d
metrics:
- type: Accuracy
value: 50.56
- task:
type: Sentence completion
dataset:
type: Muennighoff/xstory_cloze
name: XStoryCloze (hi)
config: hi
split: validation
revision: 8bb76e594b68147f1a430e86829d07189622b90d
metrics:
- type: Accuracy
value: 55.79
- task:
type: Sentence completion
dataset:
type: Muennighoff/xstory_cloze
name: XStoryCloze (id)
config: id
split: validation
revision: 8bb76e594b68147f1a430e86829d07189622b90d
metrics:
- type: Accuracy
value: 57.84
- task:
type: Sentence completion
dataset:
type: Muennighoff/xstory_cloze
name: XStoryCloze (my)
config: my
split: validation
revision: 8bb76e594b68147f1a430e86829d07189622b90d
metrics:
- type: Accuracy
value: 47.05
- task:
type: Sentence completion
dataset:
type: Muennighoff/xstory_cloze
name: XStoryCloze (ru)
config: ru
split: validation
revision: 8bb76e594b68147f1a430e86829d07189622b90d
metrics:
- type: Accuracy
value: 53.14
- task:
type: Sentence completion
dataset:
type: Muennighoff/xstory_cloze
name: XStoryCloze (sw)
config: sw
split: validation
revision: 8bb76e594b68147f1a430e86829d07189622b90d
metrics:
- type: Accuracy
value: 51.36
- task:
type: Sentence completion
dataset:
type: Muennighoff/xstory_cloze
name: XStoryCloze (te)
config: te
split: validation
revision: 8bb76e594b68147f1a430e86829d07189622b90d
metrics:
- type: Accuracy
value: 54.86
- task:
type: Sentence completion
dataset:
type: Muennighoff/xstory_cloze
name: XStoryCloze (zh)
config: zh
split: validation
revision: 8bb76e594b68147f1a430e86829d07189622b90d
metrics:
- type: Accuracy
value: 56.52
---
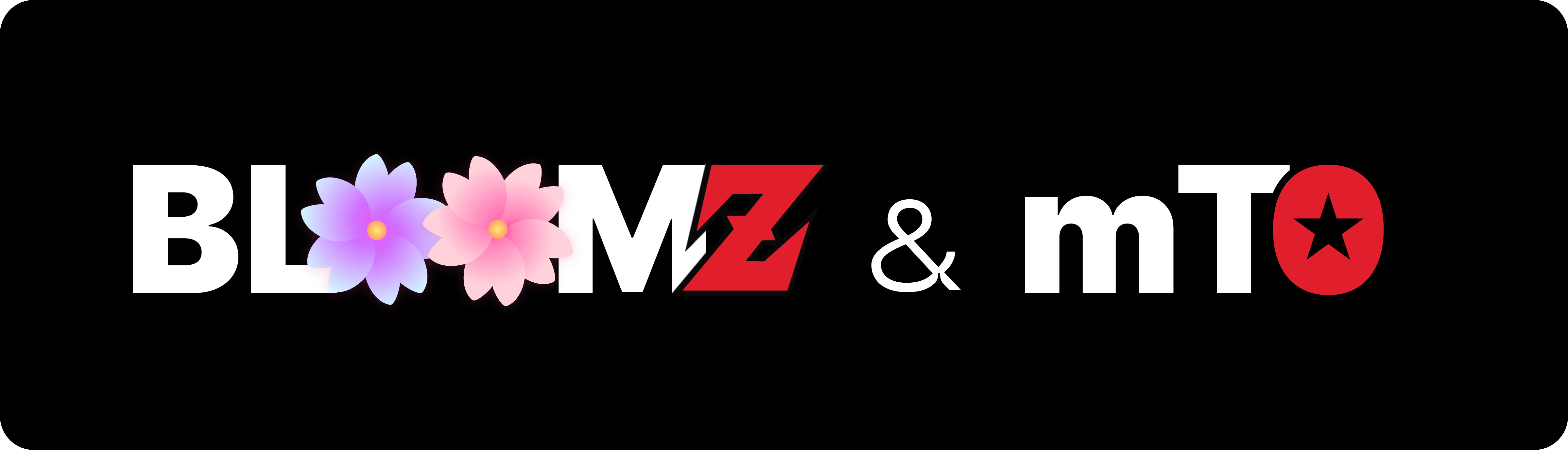
# Table of Contents
1. [Model Summary](#model-summary)
2. [Use](#use)
3. [Limitations](#limitations)
4. [Training](#training)
5. [Evaluation](#evaluation)
7. [Citation](#citation)
# Model Summary
> We present BLOOMZ & mT0, a family of models capable of following human instructions in dozens of languages zero-shot. We finetune BLOOM & mT5 pretrained multilingual language models on our crosslingual task mixture (xP3) and find the resulting models capable of crosslingual generalization to unseen tasks & languages.
- **Repository:** [bigscience-workshop/xmtf](https://github.com/bigscience-workshop/xmtf)
- **Paper:** [Crosslingual Generalization through Multitask Finetuning](https://arxiv.org/abs/2211.01786)
- **Point of Contact:** [Niklas Muennighoff](mailto:[email protected])
- **Languages:** Refer to [bloom](https://huggingface.co/bigscience/bloom) for pretraining & [xP3](https://huggingface.co/datasets/bigscience/xP3) for finetuning language proportions. It understands both pretraining & finetuning languages.
- **BLOOMZ & mT0 Model Family:**
<div class="max-w-full overflow-auto">
<table>
<tr>
<th colspan="12">Multitask finetuned on <a style="font-weight:bold" href=https://huggingface.co/datasets/bigscience/xP3>xP3</a>. Recommended for prompting in English.
</tr>
<tr>
<td>Parameters</td>
<td>300M</td>
<td>580M</td>
<td>1.2B</td>
<td>3.7B</td>
<td>13B</td>
<td>560M</td>
<td>1.1B</td>
<td>1.7B</td>
<td>3B</td>
<td>7.1B</td>
<td>176B</td>
</tr>
<tr>
<td>Finetuned Model</td>
<td><a href=https://huggingface.co/bigscience/mt0-small>mt0-small</a></td>
<td><a href=https://huggingface.co/bigscience/mt0-base>mt0-base</a></td>
<td><a href=https://huggingface.co/bigscience/mt0-large>mt0-large</a></td>
<td><a href=https://huggingface.co/bigscience/mt0-xl>mt0-xl</a></td>
<td><a href=https://huggingface.co/bigscience/mt0-xxl>mt0-xxl</a></td>
<td><a href=https://huggingface.co/bigscience/bloomz-560m>bloomz-560m</a></td>
<td><a href=https://huggingface.co/bigscience/bloomz-1b1>bloomz-1b1</a></td>
<td><a href=https://huggingface.co/bigscience/bloomz-1b7>bloomz-1b7</a></td>
<td><a href=https://huggingface.co/bigscience/bloomz-3b>bloomz-3b</a></td>
<td><a href=https://huggingface.co/bigscience/bloomz-7b1>bloomz-7b1</a></td>
<td><a href=https://huggingface.co/bigscience/bloomz>bloomz</a></td>
</tr>
</tr>
<tr>
<th colspan="12">Multitask finetuned on <a style="font-weight:bold" href=https://huggingface.co/datasets/bigscience/xP3mt>xP3mt</a>. Recommended for prompting in non-English.</th>
</tr>
<tr>
<td>Finetuned Model</td>
<td></td>
<td></td>
<td></td>
<td></td>
<td><a href=https://huggingface.co/bigscience/mt0-xxl-mt>mt0-xxl-mt</a></td>
<td></td>
<td></td>
<td></td>
<td></td>
<td><a href=https://huggingface.co/bigscience/bloomz-7b1-mt>bloomz-7b1-mt</a></td>
<td><a href=https://huggingface.co/bigscience/bloomz-mt>bloomz-mt</a></td>
</tr>
<th colspan="12">Multitask finetuned on <a style="font-weight:bold" href=https://huggingface.co/datasets/Muennighoff/P3>P3</a>. Released for research purposes only. Strictly inferior to above models!</th>
</tr>
<tr>
<td>Finetuned Model</td>
<td></td>
<td></td>
<td></td>
<td></td>
<td><a href=https://huggingface.co/bigscience/mt0-xxl-p3>mt0-xxl-p3</a></td>
<td></td>
<td></td>
<td></td>
<td></td>
<td><a href=https://huggingface.co/bigscience/bloomz-7b1-p3>bloomz-7b1-p3</a></td>
<td><a href=https://huggingface.co/bigscience/bloomz-p3>bloomz-p3</a></td>
</tr>
<th colspan="12">Original pretrained checkpoints. Not recommended.</th>
<tr>
<td>Pretrained Model</td>
<td><a href=https://huggingface.co/google/mt5-small>mt5-small</a></td>
<td><a href=https://huggingface.co/google/mt5-base>mt5-base</a></td>
<td><a href=https://huggingface.co/google/mt5-large>mt5-large</a></td>
<td><a href=https://huggingface.co/google/mt5-xl>mt5-xl</a></td>
<td><a href=https://huggingface.co/google/mt5-xxl>mt5-xxl</a></td>
<td><a href=https://huggingface.co/bigscience/bloom-560m>bloom-560m</a></td>
<td><a href=https://huggingface.co/bigscience/bloom-1b1>bloom-1b1</a></td>
<td><a href=https://huggingface.co/bigscience/bloom-1b7>bloom-1b7</a></td>
<td><a href=https://huggingface.co/bigscience/bloom-3b>bloom-3b</a></td>
<td><a href=https://huggingface.co/bigscience/bloom-7b1>bloom-7b1</a></td>
<td><a href=https://huggingface.co/bigscience/bloom>bloom</a></td>
</tr>
</table>
</div>
# Use
## Intended use
We recommend using the model to perform tasks expressed in natural language. For example, given the prompt "*Translate to English: Je t’aime.*", the model will most likely answer "*I love you.*". Some prompt ideas from our paper:
- 一个传奇的开端,一个不灭的神话,这不仅仅是一部电影,而是作为一个走进新时代的标签,永远彪炳史册。你认为这句话的立场是赞扬、中立还是批评?
- Suggest at least five related search terms to "Mạng neural nhân tạo".
- Write a fairy tale about a troll saving a princess from a dangerous dragon. The fairy tale is a masterpiece that has achieved praise worldwide and its moral is "Heroes Come in All Shapes and Sizes". Story (in Spanish):
- Explain in a sentence in Telugu what is backpropagation in neural networks.
**Feel free to share your generations in the Community tab!**
## How to use
### CPU
<details>
<summary> Click to expand </summary>
```python
# pip install -q transformers
from transformers import AutoModelForCausalLM, AutoTokenizer
checkpoint = "bigscience/bloomz-560m"
tokenizer = AutoTokenizer.from_pretrained(checkpoint)
model = AutoModelForCausalLM.from_pretrained(checkpoint)
inputs = tokenizer.encode("Translate to English: Je t’aime.", return_tensors="pt")
outputs = model.generate(inputs)
print(tokenizer.decode(outputs[0]))
```
</details>
### GPU
<details>
<summary> Click to expand </summary>
```python
# pip install -q transformers accelerate
from transformers import AutoModelForCausalLM, AutoTokenizer
checkpoint = "bigscience/bloomz-560m"
tokenizer = AutoTokenizer.from_pretrained(checkpoint)
model = AutoModelForCausalLM.from_pretrained(checkpoint, torch_dtype="auto", device_map="auto")
inputs = tokenizer.encode("Translate to English: Je t’aime.", return_tensors="pt").to("cuda")
outputs = model.generate(inputs)
print(tokenizer.decode(outputs[0]))
```
</details>
### GPU in 8bit
<details>
<summary> Click to expand </summary>
```python
# pip install -q transformers accelerate bitsandbytes
from transformers import AutoModelForCausalLM, AutoTokenizer
checkpoint = "bigscience/bloomz-560m"
tokenizer = AutoTokenizer.from_pretrained(checkpoint)
model = AutoModelForCausalLM.from_pretrained(checkpoint, device_map="auto", load_in_8bit=True)
inputs = tokenizer.encode("Translate to English: Je t’aime.", return_tensors="pt").to("cuda")
outputs = model.generate(inputs)
print(tokenizer.decode(outputs[0]))
```
</details>
<!-- Necessary for whitespace -->
###
# Limitations
**Prompt Engineering:** The performance may vary depending on the prompt. For BLOOMZ models, we recommend making it very clear when the input stops to avoid the model trying to continue it. For example, the prompt "*Translate to English: Je t'aime*" without the full stop (.) at the end, may result in the model trying to continue the French sentence. Better prompts are e.g. "*Translate to English: Je t'aime.*", "*Translate to English: Je t'aime. Translation:*" "*What is "Je t'aime." in English?*", where it is clear for the model when it should answer. Further, we recommend providing the model as much context as possible. For example, if you want it to answer in Telugu, then tell the model, e.g. "*Explain in a sentence in Telugu what is backpropagation in neural networks.*".
# Training
## Model
- **Architecture:** Same as [bloom-560m](https://huggingface.co/bigscience/bloom-560m), also refer to the `config.json` file
- **Finetuning steps:** 1750
- **Finetuning tokens:** 3.67 billion
- **Finetuning layout:** 1x pipeline parallel, 1x tensor parallel, 1x data parallel
- **Precision:** float16
## Hardware
- **CPUs:** AMD CPUs with 512GB memory per node
- **GPUs:** 64 A100 80GB GPUs with 8 GPUs per node (8 nodes) using NVLink 4 inter-gpu connects, 4 OmniPath links
- **Communication:** NCCL-communications network with a fully dedicated subnet
## Software
- **Orchestration:** [Megatron-DeepSpeed](https://github.com/bigscience-workshop/Megatron-DeepSpeed)
- **Optimizer & parallelism:** [DeepSpeed](https://github.com/microsoft/DeepSpeed)
- **Neural networks:** [PyTorch](https://github.com/pytorch/pytorch) (pytorch-1.11 w/ CUDA-11.5)
- **FP16 if applicable:** [apex](https://github.com/NVIDIA/apex)
# Evaluation
We refer to Table 7 from our [paper](https://arxiv.org/abs/2211.01786) & [bigscience/evaluation-results](https://huggingface.co/datasets/bigscience/evaluation-results) for zero-shot results on unseen tasks. The sidebar reports zero-shot performance of the best prompt per dataset config.
# Citation
```bibtex
@article{muennighoff2022crosslingual,
title={Crosslingual generalization through multitask finetuning},
author={Muennighoff, Niklas and Wang, Thomas and Sutawika, Lintang and Roberts, Adam and Biderman, Stella and Scao, Teven Le and Bari, M Saiful and Shen, Sheng and Yong, Zheng-Xin and Schoelkopf, Hailey and others},
journal={arXiv preprint arXiv:2211.01786},
year={2022}
}
``` |
nesaorg/fc_12 | nesaorg | "2024-08-14T12:57:13" | 15,558,143 | 0 | null | [
"safetensors",
"model_hub_mixin",
"pytorch_model_hub_mixin",
"region:us"
] | null | "2024-08-14T12:57:01" | ---
tags:
- model_hub_mixin
- pytorch_model_hub_mixin
---
This model has been pushed to the Hub using the [PytorchModelHubMixin](https://huggingface.co/docs/huggingface_hub/package_reference/mixins#huggingface_hub.PyTorchModelHubMixin) integration:
- Library: [More Information Needed]
- Docs: [More Information Needed] |
Dataset Card for Hugging Face Hub Model Cards
This datasets consists of model cards for models hosted on the Hugging Face Hub. The model cards are created by the community and provide information about the model, its performance, its intended uses, and more. This dataset is updated on a daily basis and includes publicly available models on the Hugging Face Hub.
This dataset is made available to help support users wanting to work with a large number of Model Cards from the Hub. We hope that this dataset will help support research in the area of Model Cards and their use but the format of this dataset may not be useful for all use cases. If there are other features that you would like to see included in this dataset, please open a new discussion.
Dataset Details
Uses
There are a number of potential uses for this dataset including:
- text mining to find common themes in model cards
- analysis of the model card format/content
- topic modelling of model cards
- analysis of the model card metadata
- training language models on model cards
Out-of-Scope Use
[More Information Needed]
Dataset Structure
This dataset has a single split.
Dataset Creation
Curation Rationale
The dataset was created to assist people in working with model cards. In particular it was created to support research in the area of model cards and their use. It is possible to use the Hugging Face Hub API or client library to download model cards and this option may be preferable if you have a very specific use case or require a different format.
Source Data
The source data is README.md
files for models hosted on the Hugging Face Hub. We do not include any other supplementary files that may be included in the model card directory.
Data Collection and Processing
The data is downloaded using a CRON job on a daily basis.
Who are the source data producers?
The source data producers are the creators of the model cards on the Hugging Face Hub. This includes a broad variety of people from the community ranging from large companies to individual researchers. We do not gather any information about who created the model card in this repository although this information can be gathered from the Hugging Face Hub API.
Annotations [optional]
There are no additional annotations in this dataset beyond the model card content.
Annotation process
N/A
Who are the annotators?
N/A
Personal and Sensitive Information
We make no effort to anonymize the data. Whilst we don't expect the majority of model cards to contain personal or sensitive information, it is possible that some model cards may contain this information. Model cards may also link to websites or email addresses.
Bias, Risks, and Limitations
Model cards are created by the community and we do not have any control over the content of the model cards. We do not review the content of the model cards and we do not make any claims about the accuracy of the information in the model cards. Some model cards will themselves discuss bias and sometimes this is done by providing examples of bias in either the training data or the responses provided by the model. As a result this dataset may contain examples of bias.
Whilst we do not directly download any images linked to in the model cards, some model cards may include images. Some of these images may not be suitable for all audiences.
Recommendations
Users should be made aware of the risks, biases and limitations of the dataset. More information needed for further recommendations.
Citation
No formal citation is required for this dataset but if you use this dataset in your work, please include a link to this dataset page.
Dataset Card Authors
Dataset Card Contact
- Downloads last month
- 210